Abstract
Current hospital vital sign monitoring for hematology and oncology patients relies on intermittent checks, potentially delaying detection of complications. Wearable devices offer continuous monitoring, promising earlier intervention. However, integration of wearable devices requires addressing user acceptance concerns. This integrative review aims to identify and address implementation barriers hindering the successful implementation of wearable devices for vital sign monitoring amongst hematology and oncology patients in acute hospital settings, and evaluate their complication detection performance. Databases such as PubMed, Cochrane, ProQuest, and IEEE Xplore, grey literature from Google Scholar, and handsearching were used to identify articles describing oncology or hematology patients above 18 years old undergoing treatment in a hospital or in-patient setting that used wearable devices for vital sign monitoring, and published from January 1, 2013–September 5, 2023. Eighteen articles fulfilled the eligibility criteria. Whittemore and Knafl’s framework guided the review. The selected articles underwent quantitative and qualitative analysis guided by the Unified Theory of Acceptance and Use of Technology framework. High adherence rates inflated user acceptance due to methodological inconsistencies. Challenges in implementation encompassed technical aspects, user-related factors, and compatibility with healthcare system workflow. While wearable devices demonstrated moderate-to-high accuracy in detecting complications, integration with non-wearable device measurements was common. Future directions include understanding healthcare professionals’ perspectives, establishing standardized procedures, developing targeted training to optimize integration into clinical workflows, and exploring the feasibility of transitioning to exclusively wearable device data for reliable complication detection. Further research is needed to address implementation barriers and optimize their use in hematology and oncology care.
Key Points
1. Wearable devices offer continuous vital sign monitoring for hospitalized hematology and oncology patients, potentially enabling earlier detection of associated complications. While studies suggest that patients generally accept wearable devices, adherence rates might be inflated due to methodological inconsistencies.2. Challenges remain in integrating these devices into clinical workflow. These include user comfort, data compatibility with electronic medical records, healthcare professionals’ acceptance, and ensuring data accuracy.
3. Further research is needed to standardize protocols for using wearable devices in this setting, explore their effectiveness for monitoring a wider range of common complications, and improve data analysis for clinical reliance on this information.
INTRODUCTION
Traditional vital sign monitoring in acute hospital settings relies on sporadic checks, posing significant challenges for hematological and oncological patients. This monitoring gap leads to delayed identification and treatment of complications like infections, neutropenic fever, sepsis, and cytokine release syndrome (CRS). These complications are more prevalent in immunocompromised patients receiving treatments like chemotherapy, radiation therapy, chimeric antigen receptor T cell (CAR-T) therapy, or stem cell transplants.1-4
Recent advancements in wearable technology can fill this gap by providing real-time, continuous monitoring of vital signs. This enables earlier detection and intervention for complications, thereby improving patient outcomes.5 These devices come in various forms, including adhesive patches that adhere to the skin (e.g., TempTraq®, Blue Spark Technologies Inc., Westlake, Ohio, USA; VitalPatch®, VitalConnect, San Jose, California, USA), biosensors that can be worn on the wrist, bicep, or other areas of the body (e.g., Fitbit, Google, Mountain View, California, USA; Everion, Biovotion AG, Zürich, Switzerland; and Garmin, Garmin Ltd., Olathe, Kansas, USA), and even smartwatches with advanced health monitoring capabilities (e.g., Apple Watch, Apple, Cupertino, California, USA; Microsoft Band, Microsoft, Redmond, Washington, USA). However, successful integration of wearable devices into the clinical workflow requires overcoming challenges like connectivity issues, alarm fatigue, user acceptance, and sifting through the constant stream of data generated by these devices. Determining what information is clinically relevant and establishing efficient workflows for clinical staff to review these reports are crucial hurdles that need to be addressed.5,6
Several literature reviews have explored the potential applications of wearable devices in an oncology or hematology context, focusing on physical activity or solely on patient adherence.7-9 However, a comprehensive assessment of their specific role in vital sign monitoring, and its impact on hematology and oncology patients, remains elusive. These reviews often overlook the unique challenges faced by this patient population and the applicability of the Unified Theory of Acceptance and Use of Technology (UTAUT) model to successfully integrate wearable devices in this context. Hence, this integrative review aims to identify and address implementation barriers hindering the successful implementation of wearable electronic devices for vital signs monitoring in hematology and oncology patients in acute hospital settings and evaluate their complication detection performance. The findings from this review will guide healthcare professionals, administrators, and policymakers on the optimal use of wearable devices in oncology and hematology in the acute care setting.
METHODS
This integrative review was performed following a five-step framework by Whittmore and Knafl: 1) problem identification, 2) literature search, 3) data evaluation, 4) data analysis, and 5) presentation.10 This integrative review aligns with the guidelines established in the Preferred Reporting Items for Systematic Reviews and Meta-analyses (PRISMA) statement.11
Literature Search
A preliminary search of PubMed clinical queries and the Cochrane Database of Systematic Reviews (CDSR) was first conducted using search terms such as “oncology,” “haematology,” “wearable”, and “hospital” or “in-patient”, but no reviews on this specific topic were identified. The gaps in similar systematic reviews were evaluated previously. Adhering to the Peer Review of Electronic Search Strategies (PRESS) checklist, text, and index terms from retrieved articles were refined and applied to all databases and grey literature.12 Published studies between January 1, 2013–September 5, 2023 were searched across accessible electronic databases, namely, PubMed, Cochrane, ProQuest, and IEEE Xplore. Sources of the unpublished studies or grey literature included Google Scholar. Lastly, a manual search was also performed to find key journals related to hematology or oncology, and wearable devices (i.e., Journal of Haematology & Oncology, Cancers Journal [MDPI], Cancer [ACS Journals], Journal of Clinical Oncology, Blood Journal, Journal of Haematology, The Lancet Oncology, JAMA Oncology, BMC Cancer, Supportive Care in Cancer Journal).
Study Selection
After the database search, all the identified records were uploaded to endnote x20, and duplicate articles were removed. Articles were included if they described oncology or hematology patients above 18 years old undergoing treatment in a hospital or in-patient setting. The wearable device must have been used on real-life hematology or oncology patients for vital sign monitoring. Potential uses of the wearable device, prototypes, and wearable devices not used for vital sign monitoring (e.g., point-of-care testing, cancer detection, physical activity monitoring, sleep quality monitoring) were excluded. Studies conducted exclusively in outpatient settings (e.g., used at home or clinic) were excluded. However, studies involving both in-patient and out-patient use were eligible. Only journal articles published in English between January 1, 2013–September 5, 2023 with full-text available were included. A pilot test was performed by the two reviewers on 10 articles to refine the eligibility criteria. Both reviewers independently screened the titles and abstracts, followed by the full texts, against the eligibility criteria. Disagreements between the reviewers at each stage of the selection process were resolved by reaching a consensus.
Quality Assessment
The Critical Appraisal Skills Programme (CASP) tool and the strengthening the Reporting of Observational Studies in Epidemiology (STROBE) checklist were used to assess the quality of the included studies.13,14
Data Abstraction
Qualitative data from patient, caregiver, and healthcare professional perspectives on wearable devices and quantitative data on accuracy performance were collected using a data collection form jointly developed by both reviewers, adapted from the JBI methodology guidance for mixed methods review.15 The two independent reviewers pilot-tested the form on five randomly selected articles, and amendments were made before its use. The two reviewers independently charted the data using microsoft excel and discrepancies were resolved through discussions.
Data Analysis
The UTAUT framework guided qualitative data analysis, allowing themes to emerge inductively. This framework comprehensively explores factors influencing wearable technology adoption in hematology and oncology care, including performance expectancy, effort expectancy, social influence, and facilitating conditions.16 Insights into potential barriers and facilitators for integrating wearable devices into clinical practice are revealed.16 Quantitative data was analysed deductively using descriptive statistics. Key findings from both analyses will be presented using tables and figures.
RESULTS
Study Selection
A total of 2,142 articles were identified from four electronic databases, grey literature, and handsearching. After removing 326 duplicate articles using endnote x20, the title and abstracts of 1,816 articles were screened for eligibility. A total of 1,735 articles were excluded as they involved trials of prototypes, did not involve vital signs monitoring, were not conducted in an inpatient setting, or were performed amongst the pediatric population.
This refined the pool to 81 articles, from which full texts were examined against the eligibility criteria. Subsequently, 60 articles were excluded for reasons such as non-primary studies; involving pediatric populations; or live presentations, meeting abstracts, and protocols without specific wearable device details or results. Consequently, 18 articles met the inclusion criteria for the final analysis. The detailed selection process is illustrated in the prisma flow diagram (Supplementary 1).
Study Characteristics
The included studies were primarily observational (n=14), conducted in non-Asian settings (n=14), and featured modest sample sizes (all <105 participants) (Supplementary 2). While the CASP checklist indicated moderate to high overall quality (Supplementary 3), the strobe checklist (Supplementary 4) revealed areas for improvement in reporting completeness. Notably, limitations in addressing potential bias, justifying sample size, and managing missing data were identified in some studies, potentially impacting the robustness and generalisability of their findings (Supplementary 3; Supplementary 4).
Wearable Device Characteristics
Wearable devices used in the 18 articles may be categorized as adhesive patches, non-adhesive biosensors, and smartwatches. Adhesive patches are distinguished by their adhesive form factor (Healthdot®, Philips, Amsterdam, the Netherlands; TempTraq; and Vitalpatch), whereas non-adhesive biosensors are primarily tailored for health monitoring purposes and lack adhesive properties (Current Health Inc., Edinburgh, UK; Everion; ViPCare SmartBand, Gadgle Creative Technology Co. Ltd., Changhua, Taiwan; Biopatch™ or BioHarness™, Zephyr, San Jose, California, USA; Fitbit Alta HR; Garmin vivosmart® 4; Fitbit Charge 2, and Garmin vivofit®). Smartwatches provide supplementary functionalities beyond health monitoring, including communication features and integration with a synchronized smartphone (Apple Watch, Microsoft Band 2). These wearable devices exhibit variations in features such as data accuracy, battery life, skin irritation risk, ease of use, comfort, monitored parameters, integration with electronic medical records (EMR), cost, and monitoring frequency (Table 1).
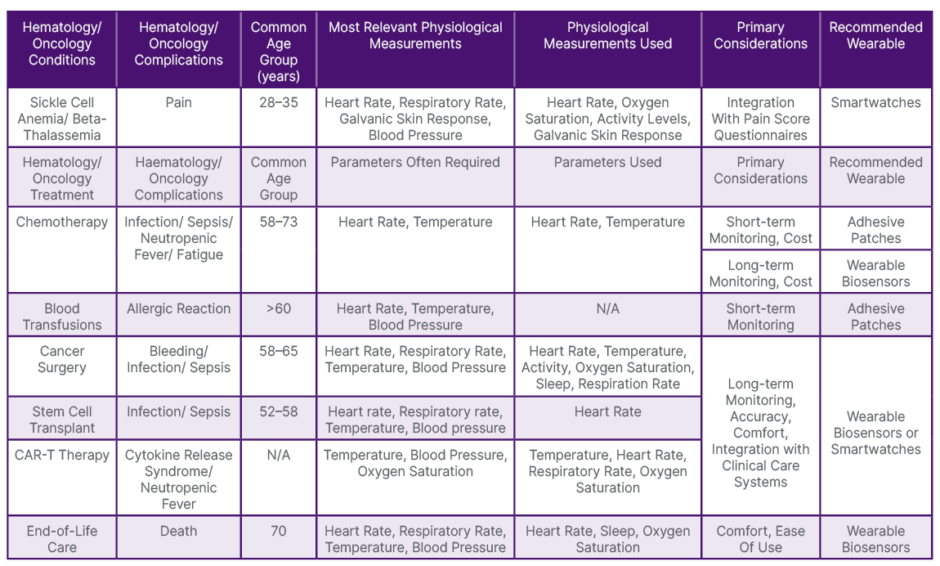
Table 1: Recommended wearable devices for hematology/oncology patients by treatment or complications (based on included articles).
N/A: not applicable.
Adhesive patches, while user-friendly with extended battery life that requires periodic replacement, tend to have lower data accuracy and have the highest likelihood of causing skin irritation.17-21 Typically employed for short-term monitoring of a single parameter, they represent a cost-effective option and are used frequently for patients undergoing chemotherapy, stem cell transplant, or blood transfusion (Table 1).17-22 Notably, TempTraq is a Food and Drug Administration (FDA)-cleared Class II medical device,18 while Biopatch is FDA-approved for electrocardiogram sensing.23
Non-adhesive biosensors, a pricier and less user-friendly alternative that requires daily recharging, provide enhanced data accuracy with a reduced risk of skin irritation. Similar to smartwatches, non-adhesive biosensors are used for long-term monitoring of multiple parameters such as post-cancer surgery, stem cell transplant, CAR-T therapy, chemotherapy, and end-of-life care.24-31
Smartwatches, albeit the most expensive and complex to operate, offer extensive functionalities and data integration with EMR. Their interactive interfaces facilitate the administration of pain score questionnaires, frequently used for patients with sickle cell anemia.32-34 Apple Watch has FDA clearance for detecting atrial fibrillation.35
Currently, only a limited number of devices (Zephyr Biopatch or BioHarness, Fitbit Charge 2, Garmin Vivo, and Apple Watch) offer simultaneous measurement of all five vital signs routinely monitored in hospitals: blood pressure, heart rate, oxygen saturation, temperature, and respiratory rate.
Adapted Unified Theory of Acceptance and Use of Technology Framework
The UTAUT framework posits that four key constructs (performance expectancy, effort expectancy, social influence, and facilitating conditions) directly influence behavioral intention and use (Table 2; Supplementary 5).
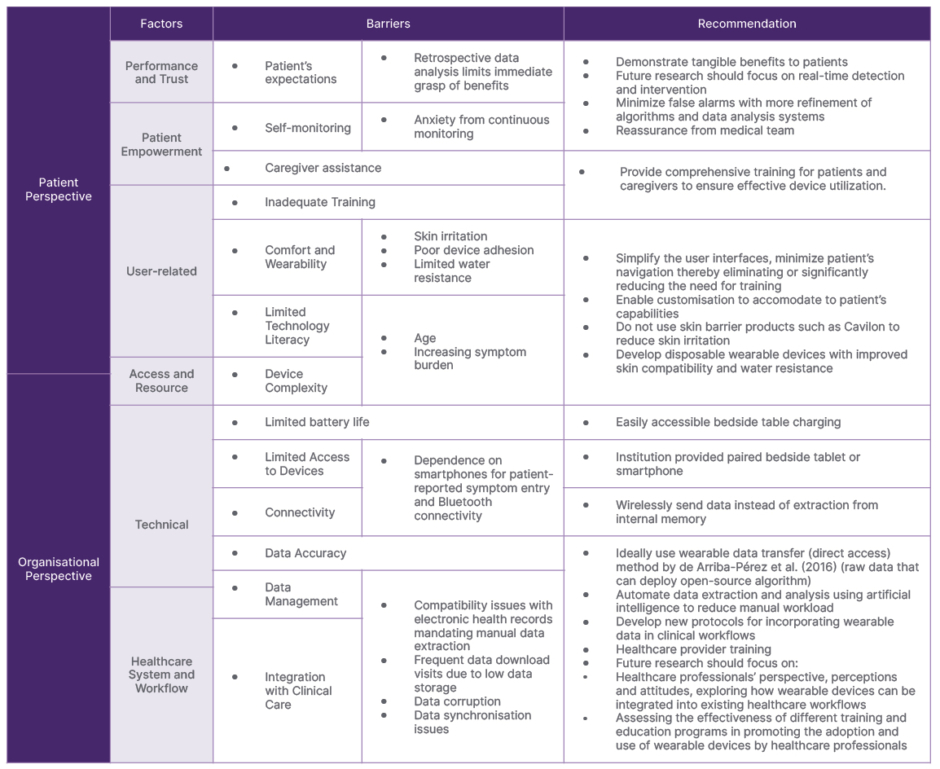
Table 2: Unified Theory of Acceptance and Use of Technology (UTAUT) to explain the successful implementation of wearables.
Performance expectancy refers to the perceived usefulness and benefits of wearable devices for both patients and healthcare professionals. While retrospective data analysis limits the immediate perception of benefits, anxiety from continuous monitoring and technical challenges further diminish the perceived value.17,26 Skin irritation, poor device adhesion, limited water resistance, and inadequate battery life, contribute to discomfort, prompting some patients to discontinue use.17,19,26,30,31 Intriguingly, despite the presumed higher technological literacy of younger patients with blood disorders, adhesive patches prevail due to shorter-term monitoring needs.
Older patients with malignancies, with a median age exceeding 53 years, grapple with device operation due to debilitating symptoms, often necessitating caregiver assistance, highlighting the importance of social influence.29,31
Caregivers, despite often being older than 65 years, exhibit proficiency in device operation after proper training. Training for patients and caregivers was noticeably absent in most studies, with only a few providing details on such initiatives.25,31,36
Effort expectancy pertains to the perceived ease of use and convenience, which is intertwined with the facilitating conditions within the healthcare system and workflow. Compatibility issues with EMR necessitating manual data extraction, frequent data download visits due to low data storage, data corruption, inadequate battery life, lack of integration into existing clinical protocols, limited data transparency due to reliance on proprietary platforms, dependence on smartphones for patient-reported symptom entry, and bluetooth connectivity, all contribute to a higher perceived effort.21,25,26 Healthcare professionals often display resistance due to data synchronization problems, lack of training, and difficulties in managing large volumes of data.21,26 Only one article offered perspectives from healthcare professionals on their experience with wearable devices.19 Despite these challenges, articles that reported patient adherence rates surpassed 70% (n=4; Supplementary 2). However, adherence definitions were heterogeneous (Supplementary 2).
Wearable Device Monitoring
Wearable devices monitor patient’s vital signs for complications such as pain, post-operative events, CRS, infection or sepsis, neutropenia, death events, and chronic, psychophysical fatigue in descending order (Supplementary 6).
Wearable Device Performance
Despite limited area-under-curve and accuracy reports (n=9), wearable devices demonstrated moderate-to-high accuracy (supplementary 6), surpassing intermittent monitoring methods.18,22,28 Van Der Stam et al.21 also found comparable performance to intensive-care-unit monitoring (Supplementary 6). However, wearable device data were often integrated with non-wearable measurements such as patient-reported outcomes and laboratory biomarkers (n=11). Studies that utilized feature selection showed that patient-reported outcome measurements were superior predictors of pain, death, and post-operative adverse events than wearable device measurements (Supplementary 6).17,24,31-34
Interestingly, while wearable devices demonstrated superior accuracy in in-patient settings, adherence rates were paradoxically higher among out-patient cohorts (Supplementary 6).27 This discrepancy suggests potential areas for improvement, particularly in enhancing adherence among in-patients, which could consequently lead to further improvements in accuracy levels. Consistent with study definitions, temperature changes remained the primary indicator for CRS, infection, sepsis, and neutropenia (Supplementary 6). Minor variations existed within definitions for the same complication, such as fever threshold for infection and sepsis (Supplementary 6). Despite inherent subjectivity, pain and fatigue were translated into objective, predictable parameters through AI and heart rate analysis.
DISCUSSION
Factors Influencing the Implementation of Wearable Devices Considerations for user acceptance
High adherence rates across studies might overestimate true acceptance due to methodological inconsistencies. Conducting semi-structured interviews is advocated to elicit a more nuanced and balanced assessment of acceptance.37 However, none of the studies utilised this method of assessing user acceptance of vital sign wearables. Frequent trial visits and Likert Scale surveys introduce potential bias.18,19,27,37 Echoing observations from Sprogis et al.,37 focusing on withdrawal reasons for user comfort can lead to reporting bias. Similarly, relying solely on adherence rates, with heterogeneous definitions across studies (Supplementary 2) limits reliable comparisons and true understanding of acceptance.38
Task-Technology Fit
Applying the Task-Technology-Fit model (alignment of device and task demands),39 key wearable features were analyzed from the included articles and tailored to patients’ conditions and treatment (Table 3), resulting in a decision tree (Figure 1) for optimal device selection.
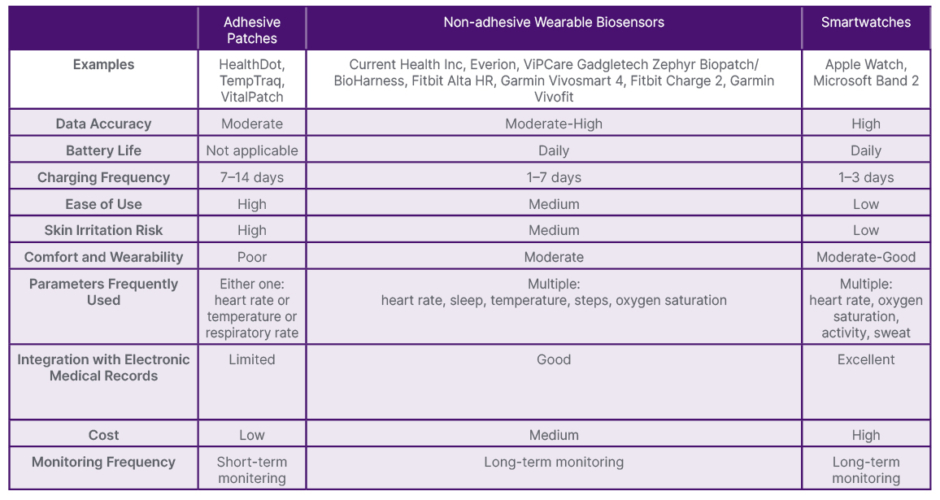
Table 3: Wearable device characteristics.
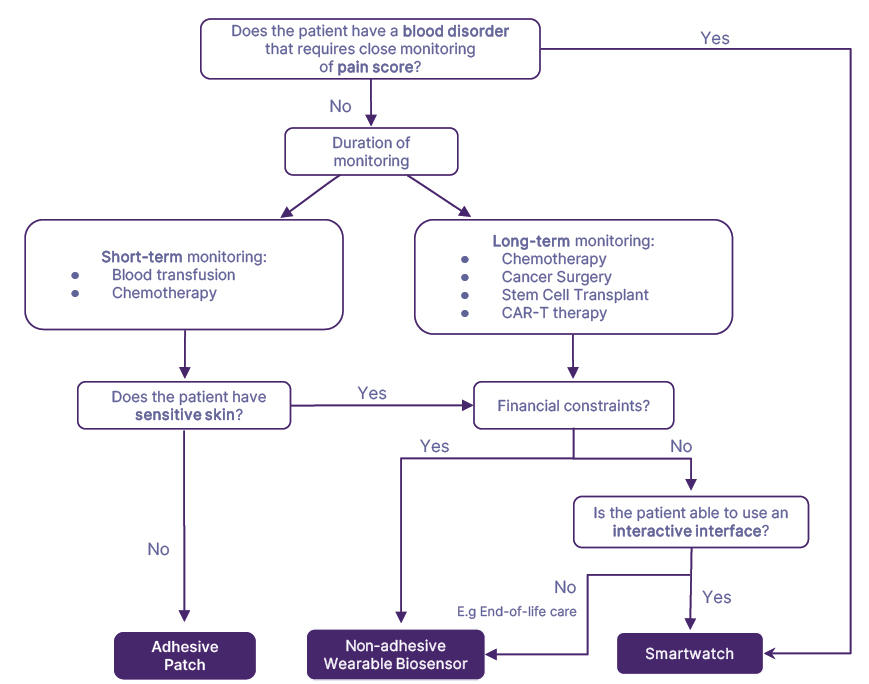
Figure 1: Decision tree for selecting wearable devices in hematology and oncology care if all forms of wearable devices contain the required physiological/ technical specifications.
CAR-T: chimeric antigen receptor T cell.
Overcoming Implementation Barriers
Beyond device selection, the Task-Technology-Fit model guides us towards alleviating implementation barriers (Table 2; Supplementary 5). Given the limitations of retrospective data analysis, future studies should explore real-time feedback systems that will facilitate earlier medical intervention, thereby enhancing patients’ perceived usefulness, and bolstering performance and trust.40 Jacobsen et al.27 demonstrated that treatment success and failure can also be tracked by wearable devices, thereby enhancing healthcare professionals’ perceived usefulness.
Mitigating patient anxiety associated with wearable devices firstly requires continuous refinement of algorithms and data analysis systems to progressively minimize false alarms, fostering trust, and reducing unnecessary distress.41,42 Secondly, proactive reassurance from the medical team is crucial. Patients need to be confident that unexpected device signals will trigger appropriate clinical escalation and that their concerns will be promptly addressed.41 Training patients and caregivers on device usage can also enhance perceived usefulness and effort expectancy.43 Alternatively, simplifying user interfaces and minimizing navigation can obviate the need for training, particularly advantageous for patients with high symptom burden or low technological literacy.44,45 Skin irritation concerns intuitively suggest the use of skin barrier products, Yet Tonino et al.19 Admonished Cavilon™ as it exacerbates irritation.
To reduce effort expectancy, non-adhesive biosensors and smartwatches should offer bedside charging options and given smartphone dependence, institutional provision of tablets or smartphones should be considered.46 Presently, the use of warehouse data transfer (indirect access) introduces vulnerabilities, risking data modifications or loss through an intermediary (smartphone application).47 Ideally, embracing wearable data transfer (direct access) would eliminate delays, providing real-time access to raw sensor data and enabling transparent deployment of open-source algorithms without relying on an intermediary device (smartphone). However, practical challenges, such as energy consumption and limited support, hinder its execution.47 Considering the impracticality of adopting wearable data transfer (direct access), the more viable alternative is wearable data transfer (indirect access), where data from wearable devices are transferred to third-party systems through an application in an intermediate smartphone. This approach, while not as ideal as direct access, overcomes the practical challenges associated with energy consumption and limited support.47
To further reduce manual workload, data extraction and analysis can be automated with AI.48 Although not explicitly stated, the absence of automatic data extraction may be due to concerns with data security and privacy.49 Therefore, data extraction methods must comply with regulatory standards.50 Limited data storage plagued Jacobsen et al.26 due to their reliance on local storage, while cloud-based storage solutions offered ample storage and enhanced security in other studies.19,20,32,51
Hilty et al.52 identified a notable scarcity of standard operating procedures and healthcare professional training pertaining to the integration of wearable devices into clinical workflows and proposed methods to teach and assess clinical competencies in this aspect.
Performance and Limitations of Wearable Device Monitoring
Although in-patient studies demonstrated high accuracy, Jacobsen et al.26 identified adherence barriers, particularly high symptom burden. Similar to Cheong et al.,53 assessing the diagnostic accuracy of wearable devices remains challenging due to the absence of true positive, false positive, true negative and false negative values; variations in reference tests; and definitions of hematological and oncological complications ([/hl]Supplementary 6[/hl]).54 Moreover, only TempTraq is medical-grade, and limited FDA clearances raise concerns about clinical dependability.18,23,35,55
Traditionally, vital signs such as temperature, heart rate, and blood pressure have been recognized as pivotal indicators of CRS, and infection or sepsis.56,57 However, due to the wearable device’s limited capability to measure solely temperature, only temperature measurements were employed for detecting these complications alongside non-wearable measurements. This constraint prompts a critical inquiry; whether the reliance on a singular parameter signifies a limitation, thereby suggesting a potential imperative to integrate additional physiological measurements from wearable devices to augment accuracy, or alternatively, a single vital sign may suffice. Subsequent research should delve into comparing the effectiveness of using only temperature versus a combination of vital signs for comprehensive detection of hematological or oncological complications.
Complications commonly experienced by hematology and oncology patients, such as nausea or vomiting, graft-versus-host disease, chemotherapy-induced peripheral neuropathy, neurotoxicity, anemia, and bleeding, remained unexamined. While measuring subjective symptoms like rash and diarrhea presents difficulties, encouraging developments exist. BioIntelliSense, Denver, Colorado, USA, demonstrates the potential for tracking vomiting, while Mantovani et al.60 shows promise in monitoring chemotherapy-induced peripheral neuropathy through gait and balance analysis from wearable devices.59,60 This opens the door for ongoing clinical assessments and trend analysis, paving the way for artificial intelligence-based monitoring.61
Nonetheless, the included articles predominantly relied on non-wearable measurements, particularly for subjective complications like pain and fatigue. Despite existing literature associating heart rate variability and blood pressure to pain aided by machine learning, and establishing links between heart rate variability and respiratory rate with fatigue, patients’ self-assessment remains the gold standard.62-64 The included articles used visual analogue scale for self-reported pain assessment.65 However, assessing non-communicative patients and accounting for memory, cognitive, and social desirability biases pose challenges.65,66 Therefore, transitioning pain assessment to measurable parameters, especially for hematology and oncology patients with high symptom burden, or nearing the end of life, who may be non-communicative, still needs to be explored.67 Further research is essential before completely replacing non-wearable measurements with solely wearable device data, particularly for developing artificial intelligence analysis models.62
Future Directions
Implementing wearable devices in hematology and oncology requires a multi-pronged approach. Tailored device selection based on individual needs, standardized procedures, and comprehensive training are key. Research must address implementation challenges, expand complication detection, and develop real-time feedback systems. Transitioning to objective pain and fatigue assessment using wearable device data and understanding healthcare professional perspectives are crucial next steps. Additionally, future studies should explore the cost-effectiveness of implementing wearable devices in these settings, considering not only device costs but also potential savings from earlier intervention and reduced hospital stays.
Limitations
The generalizability of the findings may be limited due to study characteristics. Restricted access to subscription-based databases may have imposed limitations on data acquisition.
CONCLUSION
High patient adherence suggests general acceptance of wearable devices in hematology and oncology care, but methodological variations across studies warrant caution in interpretation. Tailoring device selection to patient conditions and treatments is recommended. Challenges in implementation, including technical and user-related issues, underscore the need for further research and targeted interventions. While wearable devices demonstrate high accuracy, challenges in direct accuracy comparisons hinder comprehensive assessment. The predominant use of non-wearable measurements, aided by AI, highlights the complexity of transitioning to solely wearable device-based assessments. Complications common among these patients are underexplored, indicating a need for future investigation.