Abstract
Asthma is a complex condition with heterogeneous patterns of symptoms underpinned by different underlying pathophysiological mechanisms and treatment responses. Analyses of data from birth cohorts and patient studies, from the subjective hypothesis-testing approach to the data-driven hypothesis-generating approach, have improved the current understanding of asthma’s heterogeneity. Despite the rapid proliferation of new sources of data and increasingly sophisticated methods for data mining and revealing structure, relatively few findings have been translated into clinically actionable solutions for targeted therapeutics or improved patient care. This review focusses on why an integrated approach may be a more powerful catalyst for improved patient outcomes compared with the artificial and imposed dichotomy of hypothesis-generating versus investigator-led subjective approaches. As the factors shaping the development and control of asthma affect individuals dynamically in response to treatment or environmental factors, deeper insights can be garnered through the integration of data with human expertise and experience. The authors describe how integrative approaches may have greater power to provide a more holistic understanding of the pathophysiological mechanisms driving asthma heterogeneity, discussing some of the key methodological challenges that limit the clinical use of findings from asthma research, and highlighting how recent examples of integrative approaches are building bridges to ensure that the power of emerging sources of data, coupled with rigorous scientific scrutiny, can lead to a more nuanced understanding of asthma.
INTRODUCTION
Over the last two decades, a substantial effort has been devoted to understanding the heterogeneity of asthma.1-7 The architecture of wheezing illness during childhood has been described based on temporal patterns of symptoms using data-driven techniques applied to longitudinal data from birth cohort studies.3-5,7-10 As a consequence, the conceptual framework of asthma heterogeneity is now accepted within the clinical and research communities.1 However, the main aim of discovering ‘asthma endotypes’11 and their underlying pathophysiological mechanisms for the identification and development of novel targeted therapeutics appears as elusive as ever.
In part, progress has been stymied by the methodological and disciplinary silos. The rapid increase in the ability to generate, share, and access large amounts of data, including longitudinal clinical information and biomarkers, various ‘omics’ technologies, and environmental exposures, coupled with advances in data-driven techniques to analyse high-dimensional data, has made it, on occasion, challenging to discern what problems we are seeking to address, or how findings are relevant in a real-world setting.12 Given that big data sets may contain many thousands of variables, or differ in terms of the format or level of the data (e.g., clinical history, laboratory tests, environmental and behavioural factors, various biomarkers, proteomic data, and genome-wide genotyping), it is not possible to define a priori all possible causal and associational mechanisms. An integrated approach to research may enable the power of these resources to be harnessed in ways that translate into a better understanding of causal mechanisms, more accurate diagnoses, and more personalised treatment. The integration of data, methodologies, and human expertise to understand the results can only occur through cross-disciplinary research, with the central principle that basic scientists, geneticists, clinicians, and data scientists work together to understand the clinical heterogeneity of complex diseases and the mechanisms underpinning them.
In this review, the authors set out to describe the evolution of analytical frameworks in asthma epidemiology, from the subjective hypothesis-driven to the data-driven hypothesis-generating approaches; highlight why an integrated approach may be a more powerful catalyst for improved patient outcomes; and identify the key challenges faced by healthcare professionals in adopting findings to clinical practice.
EVOLVING FRAMEWORKS OF DATA ANALYSIS IN ASTHMA RESEARCH
Long-term follow-up in birth cohort studies has allowed a shift in emphasis in temporal perspectives from the static cross-sectional approach to a more dynamic longitudinal approach. By explicitly allowing for time in the mediation of disease development, the longitudinal approach has allowed us to establish whether individuals affected by symptoms of the disease at one point in time are the same individuals who have the disease at later time points, ascertain temporal variations across individuals in terms of the timing of onset or remission and the persistence and recurrence of episodes, and identify the risk factors that discriminate these different temporal patterns.3,10 Analytical approaches have progressed from supervised analyses testing-specific hypotheses to statistical data-driven classification techniques.3 In the former, typologies of disease or hypotheses are proposed by investigators or clinical experts, usually based on patterns of symptoms observed in a clinical situation.13 The Tucson Children Respiratory Study (TCRS) was one of the first studies to use longitudinal data to differentiate childhood wheezing phenotypes based on the presence of temporal patterns or the absence of symptoms.2 Three mutually exclusive phenotypes (transient early, late-onset, and persistent wheezing) were described from data collected at two time points (aged 3 and 6 years).2 While such studies have been instrumental in introducing and confirming the idea of heterogeneity of childhood wheezing and asthma, the subjective approach has several potential limitations. For example, there is a risk of limiting the predictive ability of a model by restricting the set of inputs, imposing a structure that does not necessarily fit the data, failing to identify groups with truly distinct patterns, and/or missing rare patterns.
In contrast, data-driven algorithms enable the analysis of large quantities of complex data for the identification of hidden patterns within such datasets. Continuous advances in computational power allow pattern discovery in high-dimensional data to take place with increasingly greater efficiency. As data-driven techniques are hypothesis-neutral, they are useful for examining heterogeneity based on distinctions that are not known a priori, and for making predictions about outcomes while remaining agnostic towards specific predictors.14 This has allowed for the discovery of patterns that could not have been predicted in advance. Numerous data-driven algorithms have been applied in asthma research. For example, latent class trajectory models, which are a class of probabilistic models in which repeated measurements of manifest symptoms are modelled in order to derive homogenous subtypes, have been extensively applied to derive distinct wheeze and lung function trajectories.4,7,8,15,16 One advantage of such methods is that objective statistical criteria are used for judging whether clusters (classes or subtypes) represent true variation in the population. Clusters discovered using data-driven approaches are not observed, but hidden, and should not be referred to as ‘phenotypes’; however, as this term has widely been used in the literature, the authors will continue to use this nomenclature.6
Discovery of wheeze phenotypes using data-driven methods is susceptible to inconsistencies with respect to the number of discovered phenotypes, the size of each class, and the labels ascribed to them.6 For example, a review, which compared wheeze phenotypes derived from latent trajectory modelling across 28 studies, found that the number of phenotypes ranged from 3–8. Another review found considerable differences in the size of ‘common’ phenotypes in different cohort studies6 (e.g., there was up to a 10-fold difference in the proportion of children classified as late-onset wheezing [3.7–35.8%]).7 The inconsistencies between studies may arise from differences in the number of data points, the length of the intervals between data collection points, the age at follow-up, and the study’s duration, sample size, population differences, and definition of the symptom17 (e.g., parentally-reported versus doctor-diagnosed).18
Bayesian analysis,9 hidden Markov models,9 and temporal clustering19 have been applied to challenge the paradigm of the atopic march, which assumes that there is a natural progression of symptoms from eczema to asthma and rhinitis. This paradigm is based on observations using cross-sectional data on population prevalence. However, modelling longitudinal data within individual patients revealed heterogenous patterns, with <7% of children with any of these symptoms following the atopic march trajectory.9 Other applications of machine-learning include Bayesian networks coupled with feature selection methods for the discovery of patterns of allergic sensitisation,20-22 principal components analysis to investigate whether syndromes of co-existing respiratory symptoms could be derived using responses to >100 questions from validated questionnaires,23 Bayesian estimation of a mixture of Bernoulli distributions to describe the architecture of IgE responses to multiple allergenic proteins during childhood,24 Gaussian mixture model to cluster human blood cell cytokine responses to rhinovirus-16,25 and the use of network analysis and hierarchical clustering to explore the connectivity structure of allergen component-specific IgE, which demonstrated that the interaction patterns of IgE rather than individual ‘informative’ components are associated with asthma.26
CHALLENGES TO BRIDGING THE GAP BETWEEN BIG DATA RESEARCH AND CLINICAL USE
Currently, there is no consensus on what the best approach should be to understand asthma heterogeneity, how best to identify distinct underlying pathophysiological mechanisms, and how to implement these findings in a clinically useful way.12 One potential flow is summarised in Figure 1.
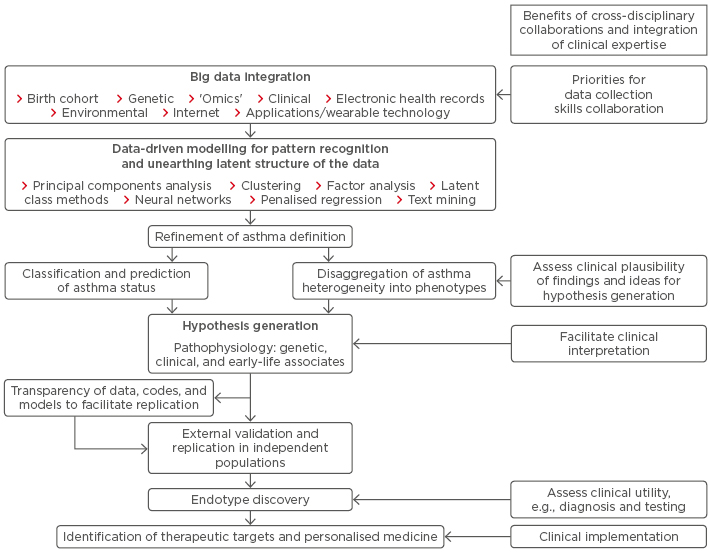
Figure 1: From asthma research to clinical implementation.
Identification of Children at High Risk of Asthma
Prediction modelling to identify individuals at a higher risk of asthma is important and was identified as the top research priority by the European Asthma Research and Innovation Partnership (EARIP).27 Several algorithms have been proposed for predicting persistent asthma in school age using early-life features, including the Asthma Prediction Index,28 the Isle of Wight score,29 the PIAMA risk score,30 and the Leicester31 and Manchester scores.32 However, these tools have not been widely adopted clinically.33 A systematic review found that these tools typically have low sensitivity and positive predictive values, making them unsuitable for the precise identification of high-risk individuals in a clinical setting.34 Given the heterogeneity of asthma, algorithms may be required to predict different ‘asthmas’ instead of a one-size-fits- all tool.35
Lack of Uniformity in Defining the Dependent Variable
Comparison of prediction tools and adoption in practice is complicated by the fact there is no uniform operational definition of asthma. This creates challenges in identifying consistent early-life predictors, genetic and environmental associates, and pathophysiologic mechanisms.34,36 A number of studies have indicated that the choice of case definition has a large impact on the estimate of asthma prevalence, as well as performance measures of predictive models. van Wonderen et al.37 found 60 different definitions of childhood asthma in cohort studies in 122 published articles.37 Applying four common definitions to a single cohort, the authors found that prevalence estimates varied from 15.1–51.1%.37 These finding have implications for comparing studies that use different definitions of asthma and suggest the importance of conducting sensitivity analyses to assess the impact of heterogeneous definitions.
Transparency of Replication of Algorithms
Clinicians require access to their patients’ data in an absorbable and reliable way that integrates seamlessly with their clinical workflow and does not detract from their key priority of providing quality care during a short patient visit. Without interpretive tools that can be readily incorporated in daily practice, there may be a risk of valuable research findings being overlooked, as actions for decision-making may not be obvious. The statistical literacy of the clinical community is not keeping pace with the proliferation of new data-driven techniques and the associated terms (e.g., negative matrix factorisation,19 probabilistic causal network analysis,38 decision trees,39 and least absolute shrinkage and selection operator [LASSO]-penalised logistic regression31). Computational transparency and reproducibility of research findings are increasingly complicated by the density and complexity of the code underlying models implemented using a variety of programming languages.40 Such issues are increasingly being recognised, with organisations, including Fairness, Accountability, and Transparency in Machine Learning, calling for greater awareness, debate, and research on such issues. Recently, practical solutions have been proposed, such as a toolkit for enhanced transparency, which includes the use of open-source software, documentation of analyses steps, data archiving, and version control of code using web-based hosting services, such as GitHub, Inc., San Francisco, California, USA.41 Timely syntheses of findings from the growing research output can help clinicians to understand research with a potential for clinical application. For example, Pecak et al.42 recently developed a catalogue of 190 potential asthma biomarkers from 73 studies covering 13 omics platforms (including genomics, epigenomics, transcriptomics, and proteomics).42 They identified 10 candidate genes linked to asthma that were present in at least two omics levels, thus demonstrating the potential for prioritising specific biomarker research and the development of targeted therapeutics.
FUTURE DIRECTIONS: TAKING AN INTEGRATIVE APPROACH
Integrating Data
The proliferation of new data types coupled with advances in computational power may offer new opportunities for integrating different data sources to understand common complex diseases more holistically. Recent advances in molecular techniques offer promising opportunities to disentangle phenotypic characteristics that reflect underlying pathological mechanisms.43 In this context, systems biology is an approach that investigates organisms as integrated systems comprising dynamic and interrelated genetic, protein, metabolic, and cellular components. Combined with mathematical, bioinformatic, and computational techniques, systems biology can help to elucidate the directionality of relationships between variables at a more holistic level, thereby moving away from associative to more causal analyses.38,44 In the longer term, findings from such data have the potential for the development of non-invasive and quick diagnostic assessments for use in clinics.45-47 With the birth of genome-wide association studies (GWAS), researchers are able to investigate the relationship between hundreds of thousands of genetic markers with a phenotype.48 However, most large GWAS in the field of asthma use the broadest possible definition of the primary outcome (e.g., ‘doctor-diagnosed asthma’). In contrast, using deep phenotyping, a recent comparatively small GWAS discovered the association of a specific asthma phenotype (early-life onset with severe exacerbations) with a functional variant in a novel susceptibility gene CDHR3 (rs6967330).49 This SNP was not associated with doctor-diagnosed asthma in any of the large-scale GWAS. Subsequent in silico studies have shown that rs6967330 mediates rhinovirus-C binding and replication, and that a coding SNP in CDHR3 mediates enhanced rhinovirus-C binding and increased progeny yields.50 Several companies are currently pursuing this as a therapeutic target. This example shows the potential of moving from much better phenotyping to genetic association studies, discovery of mechanisms through functional studies, and the identification of therapeutic targets for tailored clinical treatment. Figure 2 summarises this desired sequence.
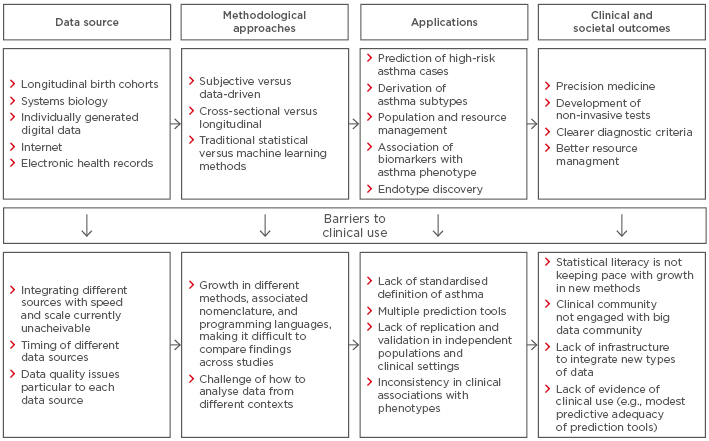
Figure 2: Barriers to clinical implementation of asthma research.
New possibilities for asthma research are also emerging from personally tracked data from the ubiquitous use of digital devices. Data from Google, Twitter, and Facebook have made real-time information about daily behaviours, health status, and geographical locations widely accessible on an unprecedented scale. The potential for using web-based data for surveillance of trends has been demonstrated in other diseases, such as flu,51 lupus,52 and multiple sclerosis.53 In contrast, traditional sources of surveillance data are based on a time lag, which makes prompt responses infeasible. Real-time models could help healthcare facilities anticipate asthma-related visits and hospitalisations, and plan staffing and resource management in areas of high risk. A recent study has capitalised on the use of online data to demonstrate the potential for asthma surveillance.54 Text mining was used to link asthma-related tweets with electronic medical records using geolocation data, along with near real-time environmental data from an air quality sensor. When the number of asthma-related tweets increased in a particular week, the number of asthma emergency department visits or hospitalisations increased proportionally during the following week. The predictive model suggested patterns of accident and emergency visits with around 75% accuracy.
Individually generated data are also emerging from synergies between medical technology and smartphones. Bluetooth-enabled smart inhalers and peak flow meters55,56 allow individuals to monitor lung function, medication use, and severity of symptoms. myAirCoach, which is a pan-European Union (EU) consortium comprising patient groups, academic institutions, and technology and pharmaceutical companies, aims to provide an evidence base for the benefits of integrating sensor technology with computational modelling to provide personalised feedback to patients on how to manage their condition daily.57,58 The use of such data may provide clinicians with warnings on exacerbations, which would allow them to tailor medication accordingly.
Table 1 summarises the strengths and limitations of different sources of data.3,10,38,42,56-64 These types of data have the potential to uncover different aspects of asthma heterogeneity with greater granularity and certainty, but they are a complement to, rather than a substitute for, traditional or other forms of data.
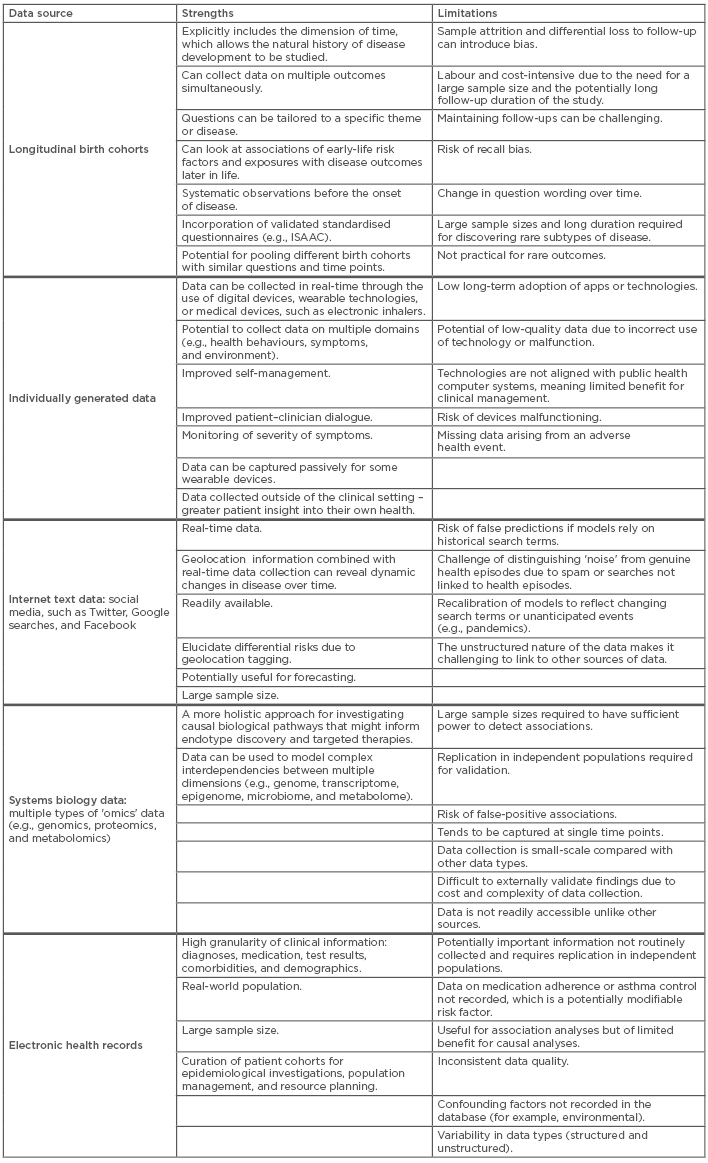
Table 1: Examples of asthma data sources with associated strengths and limitations.
ISAAC: The International Study of Asthma and Allergies in Childhood.
Integrating Multidisciplinary Expertise
One potential risk of ‘allowing the data to speak for itself’ is that data analysis may become divorced from rigorous scientific scrutiny and meaningful clinical interpretation.12 The use of modern techniques, such as machine learning, does not and should not preclude the use of more traditional statistical hypotheses-testing approaches.14,20,25 The patterns can be discovered in large and heterogeneous data, yet clinical and basic science domain experts can guide formulation of new hypotheses and provide interpretation to findings.59 For example, a recent study, which applied latent profile analysis to the Tasmanian Longitudinal Health Study, identified six discrete lung function trajectories,16 five of which were remarkably similar to trajectories from pre-school age to early adulthood in two UK birth cohorts.15 Using logistic regression, the study found that three of these trajectories were associated with childhood asthma, and the same trajectories were also associated with chronic obstructive pulmonary disease in later life, suggesting that early-life risk factors could lead to poorer lung growth and adult risk factors could accelerate lung function decline.
As the number of relationships being tested increases, there is a risk of identifying false-positive associations in the absence of previous guidance about the clinical plausibility of such findings.65 Big data can only explain part of the picture, and clinicians can provide a more contextualised understanding through their experience, knowledge of detailed clinical histories, and being able to explain variations across their patients.66 Experts can review the findings from big data studies, which may generate promising leads for further enquiry.
An integrated approach to big data may enable us to harness the power of big data in ways that translate into a better understanding of causal mechanisms, more accurate diagnoses, and more personalised treatment. Integration can occur at different levels through cross-disciplinary research (for example, the Study Team for Early Life Asthma Research [STELAR] consortium,60 MedALL,67 U-BIOPRED,68 Breathing Together consortium69), wherein basic scientists, geneticists, clinicians, and data scientists work together to understand the mechanisms of relevance to clinical heterogeneity of asthma. Another way of bridging the divide between the clinical and big data communities is to understand the tools clinicians need to improve outcomes for their patients by taking a ‘team science’ approach. As an example, a recent pan-EU consensus exercise led by the EARIP sought to identify key areas for research funding that would, most likely, improve asthma diagnosis and patient care.27 Experts comprised patients, patient organisations, healthcare professionals, researchers, industry representatives, and policy influencers. The prediction of asthma in preschool children with reasonable accuracy, how to integrate new biomarkers (such as genomics, proteomics, and metabolomics) in the diagnosis and monitoring of asthma, and the measurement of exhaled volatile organic compounds were identified as priority areas for research. This demonstrates how integrating multidisciplinary expertise has the potential to inform research, and for findings to be translated into improved outcomes for patient care.
CONCLUSION
One of the goals of asthma research is to understand disease heterogeneity with the aim of providing personalised treatment. There needs to be a shift away from the artificial dichotomy of data-driven hypothesis-generating versus more traditional hypothesis testing approaches towards a more integrated one, whereby cross-disciplinary collaborations can facilitate rigorous scientific scrutiny and interpretation of findings. No single source of data can uncover the complex dynamics driving asthma heterogeneity, and triangulation (integration of evidence from several different approaches with differing and unrelated sources of bias) is critically important to fill current knowledge gaps and improve causal inference.70,71 With the advent of new and exciting sources of data, there is huge potential for integrating these to provide a more holistic understanding of the disease at a very personal level. As the factors shaping the development and control of asthma affect individuals dynamically in response to treatment or environmental factors, deeper insights can be garnered through integration. Knowing in real-time when and where symptoms are exacerbated, in combination with refined subtypes and environmental data, may help identify personal triggers and inform a personally tailored care plan.
Research needs to take greater steps to demonstrate clinical utility, or it risks being consigned to research for research’s sake. Tools need to be developed that clinicians can integrate into daily practice to make decision-making more efficient and personalised. Steps need to be taken to improve the statistical literacy of healthcare professionals through greater education to bridge the divide with the big data industry. It is essential that clinicians engage in debates surrounding big data and healthcare as a step towards breaking down the siloed approach.