INTRODUCTION AND OBJECTIVE
Bladder tumours have a high intravesical recurrence rate after transurethral resection of the bladder tumour (TURBT).1,2 Overlooking tumours during TURBT occasionally causes early intravesical recurrences. In particular, flat tumours are sometimes overlooked because those tumours are more difficult to detect than elevated papillary tumours.3 Detecting flat tumours is clinically beneficial because those tumours represent carcinoma in situ and are high-risk tumours amongst bladder tumours. Recently, AI has been developed as a new diagnosis system. You Only Look Once (YOLO) is a fast object detection AI system,4 and this is a deep neural network that detects objects in an image. This technique has the potential to improve flat tumour detection rate in cystoscopy; however, only a few studies reported the utility of AI for detecting flat tumours in cystoscopy. In this study, the authors aimed to develop an AI system to detect flat tumours in cystoscopic images.
MATERIALS AND METHODS
The authors constructed an object detection system based on YOLO. They obtained cystoscopic images of bladder tumours during TURBT procedures at their institutions between December 2019–September 2021. Those cystoscopic images obtained were divided into training and testing data in an 8:2 ratio. YOLO was trained to detect bladder tumours in an image by learning the tumour features from training data. AI testing was conducted using YOLO, and its sensitivity, specificity, and area under the receiver operating characteristic curve were evaluated for flat tumour images.
RESULTS
The authors obtained 2,371 tumour images and 254 non-tumour images. In tumour images, 436 flat tumour images were included. They used 1,896 images for the AI training and assessed the accuracies of the AI system. The sensitivity and specificity were 90.0% and 90.6%, respectively. With a likelihood score of 0.163, the area under the receiver operating characteristic curve in detecting flat tumours was 93.4%. An example of the detection is shown in Figure 1.
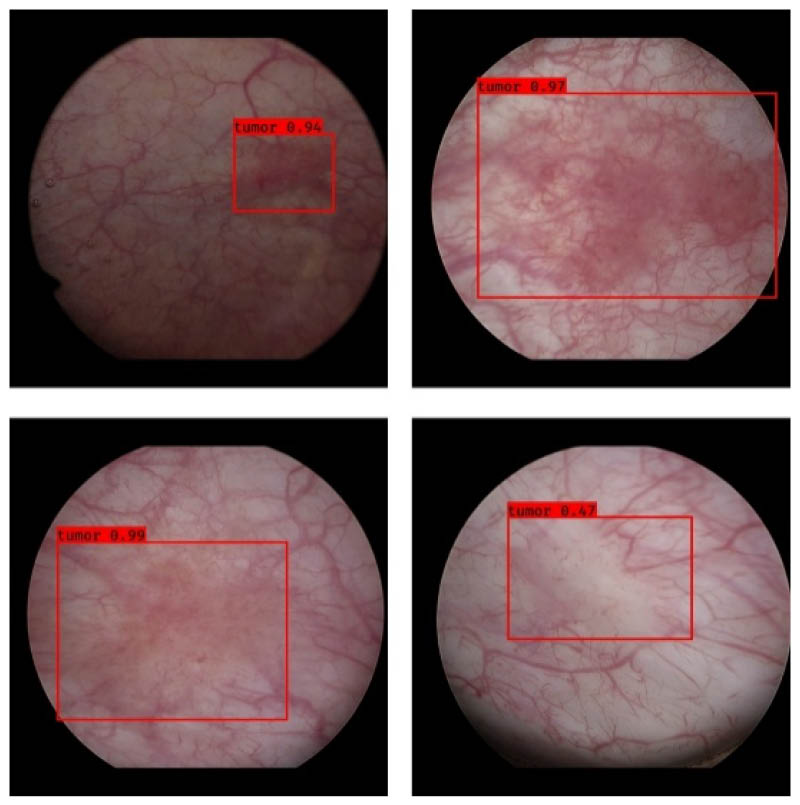
Figure 1: Representative flat tumour detection by You Only Look Once.
The authors’ proposed AI system could detect small and large flat bladder tumours. The lower right of images represents the whiteness lesion that was a carcinoma in situ, and their proposed AI could detect this flat lesion.
CONCLUSION
The system proposed by the authors can detect flat bladder tumours in cystoscopic images. This system has the possibility to improve detection accuracy during TURBT and might be beneficial to reduce the recurrence rate of bladder tumours after TURBT in the future.