Abstract
Precision medicine is quickly emerging as an exciting new medical model in which patient information is extracted from their lifestyle, environmental, and genetic data. These data will be used to augment and refine traditional medical data to provide a higher level of specificity for disease prevention and patient care. Of the three pillars supporting precision medicine, this paper takes a deeper look at the genetic and genomic pillar; in particular, investigating the role the field of ‘omics’ has played in helping to develop precision medicine. The term omics is used to describe the collective research efforts of molecular biology for various subdomains (e.g., genomics, proteomics, metabolomics). While this paper is not exhaustive in scope, cases where omics has impacted both clinical practice and public health are highlighted, as well as a discussion of where omics has yet to bridge the gap between these two areas of medicine. The aim of this manuscript is to provide the reader with insight on the particular challenges and benefits of pursuing precision medicine.
INTRODUCTION
Precision medicine is a medical model in which an individual’s genes, environment, and lifestyle are used as additional layers of patient data in disease treatment and prevention plans (Figure 1).1 Diagnostic clustering and categorisation of patients via parameters such as genetics, biomarkers, phenotypes, and psychosocial characteristics will enhance the level of care physicians will be able to provide.2 This concept is not new and, in fact, the model has already obtained several monikers, like personalised and individualised medicine.3,4 Linking an individual’s environmental exposure to the associated health impact has given rise to the study of the exposome5 and the field of molecular pathological epidemiology (MPE).6 The depth and breadth of the exposome and MPE studies eclipse many aspects of the other two pillars. For example, MPE research probes the complex network of macroenvironments with tissue microenvironments, and the unique profile creates multiple factors, such as microbiomes, transcriptomes, and interactomes, to name only a few.7 This paper explores the genomic pillar of precision medicine to help gauge the model’s overall progress; however, this should not be taken as emphasis of its importance over the environmental and lifestyle components, which are equally valuable.
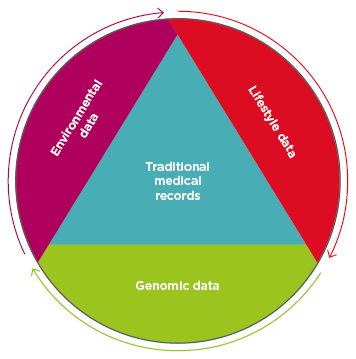
Figure 1: Precision medicine adds three additional data sets to existing traditional patient medical records: data about the patient’s environmental exposures, their lifestyle, and their genomic data.
Announced in 2015, the USA-based Precision Medicine Initiative (PMI) established $215 million to help actualise precision medicine.8 To help drive this research initiative, the National Institutes of Health (NIH) aims to build a voluntary research cohort of ≥1 million people.9 A wide range of data types will be collected from each participant in this cohort, including genes and microbiome sequencing, lifestyle data, metabolites, and wearable sensor data. As of June 2017, beta testing for this programme, now known as ‘All of Us’, has begun.10
How close is precision medicine to reality? Is it merely years, or closer to decades, away? This paper investigates some of the impact human genome, proteome, and metabolome research has had on clinical practice. These fields of research are colloquially known as the ‘omics’, designated as such for the suffix used to identify the biology research focus (e.g., genomics, proteomics, metabolomics). How do we maximise efforts in these areas? This paper aims to leave the reader with a greater understanding of how omics is shaping precision medicine, the promise it provides, and an appreciation for the amount of effort still required. Cases of omics impacting either clinical practice or public health, in addition to conditions which omics has yet to bridge the final gap from clinical practice to public health, are highlighted.
The PMI aims to: “Pioneer a new model of patient-powered research that promises to accelerate biomedical discoveries and provide clinicians with new tools, knowledge, and therapies to select which treatments will work best for which patients.”11 To provide an accurate overview of what has been achieved by omics and what is still a work in progress, two different cases of omics use are examined. The first case reviews the enhancements omics have provided to health surveillance systems and the second case inspects cancer research, which was included as a major target of the PMI, as a part of the Cancer Moonshot initiative.
Health surveillance using genetic sequencing for pathogens has direct applications for both fields, whereas omics and cancer is just starting to yield results in clinical practice but remains largely on the drawing board for public health.
HEALTH SURVEILLANCE SYSTEMS
A powerful tool for public health has been surveillance systems enhancements. These systems incorporate biomedical data to provide continuous analysis and interpretation in a systematic way. The Centers for Disease Control and Prevention (CDC) and World Health Organization (WHO) both operate surveillance systems that help to watch for pathogens with the potential of becoming widespread epidemics. DNA sequencing has had a profound impact on global public health surveillance efforts; sequencing is not just a tool that is effective in identifying pathogens, but it is useful in efforts to trace an infectious agent’s origins and provide insight on how best to combat the pathogen. These discoveries can then be sent back to the ‘front lines’ of clinics, hospitals, or triage centres in the event of an actual outbreak. In 2009, sequencing was a major component in tracing lineages of the A(H1N1) influenza virus, also known as swine flu. By performing analysis of A(H1N1), previously unrecognised molecular determinants were detected and were suspected to be involved with the increased virility of the pathogen.12 Similar techniques were repeated for the Ebola virus (EBOV) in 2014. A cohort of 78 patients from Sierra Leone provided 99 EBOV genomes for sequencing; this method identified that the West African variant of EBOV most likely diverged from a central African lineage in 2004.13 This new subtype then spread from Guinea to Sierra Leone in May 2014.13 This information helped to shape the efforts of vaccine development, therapies, and diagnostics occurring in the field and these techniques have also been deployed against the Zika virus (ZIKV).14 Efforts for sequencing ZIKV have been staged by collecting amniotic fluid samples from patients with fetuses diagnosed with microcephaly.15 Sequencing of 110 ZIKV genomes was recently used to track the evolution and spread of the virus from Brazil into Central America and into the USA.16 Work on ZIKV is still developing, but it provides a good example of how deeply embedded omics techniques have become for public health and how the knowledge gained is transferred to clinical practice.
DEVELOPMENT OF PERSONALISED CANCER TREATMENTS
Personal cancer therapy strategies provide a strong illustration of omics in action, from the targeting of BCR-ABL mutations with imatinib for patients suffering from chronic myeloid leukaemia,17 and the use of crizotinib to assist lung cancer cases where the patient has the EML4-ALK fusion gene.18 One can also look at the Icahn School of Medicine at Mount Sinai’s Institute for Genomics and Multiscale Biology personalised cancer treatment programme. In 2013, they transplanted tumour cells from a colon cancer patient into fruit flies.19 The use of this fly model allowed for rapid propagation of the cancer cells thanks to the accelerated lifecycle of the flies. By taking this approach, a multitude of cancer drugs could be tested quickly against the cells from the flies to find the most efficacious drug for targeting the patient’s tumour cells.19 In an additional cancer study, the researchers opted to not use genome-wide association studies (GWAS) and instead performed whole exome sequencing (WES); WES is a more focussed method in comparison to GWAS, targeting only protein coding sections (exons) and skipping the non-coding sections (introns). This method also reduces costs and allows for comparison across a large population. WES was performed for 46 patients and samples were genotyped using single nucleotide polymorphism microarrays for both tumour and patient-matched normal tissue.20 RNA sequencing was performed to find somatic (tumour-specific) mutations, copy number alterations, gene expression changes, gene fusions, and germline variants.20 The results of this study showed 17.3 cancer-related somatic mutations per patient.20 To benchmark results, samples were also run against Ion AmpliSeqTM Cancer Hotspot Panel v2 (CHPv2) (Thermo Fisher Scientific, Waltham, Massachusetts, USA), a sequencing assay used to find somatic mutations.21 Results were also compared to other somatic mutation detection tools, Oncomine® Cancer Research Panel (OCP) (Thermo Fisher Scientific)22 and FoundationOne® (Foundation Medicine, Cambridge, Massachusetts, USA),23 and the results were found to be 13.3-fold, 6.9-fold, and 4.7-fold improvements, respectively, against the other somatic tests. This investigation was able to identify genetic drivers and helped determine the best-fit therapeutic options. Medically actionable options were identified in 91% of the patients, even with a wide range of cancers being surveyed (colorectal, breast, thyroid, multiple primaries, and unknown primary), and 28% of the cancers had already metastasised. These results are very promising, and the study led to adjustments to the treatment plans for four of the participants.
GENOME-WIDE ASSOCIATION STUDIES IN PUBLIC HEALTH INFORMATICS
Next-generation sequencing is providing the means to perform whole genome sequencing, which has been a paradigm shift for how we can address complex diseases.24 This provides a new depth of insight for individuals, but for public health informatics, the interest in populations over patients changes a few parameters. Public health informatics uses GWAS as a screening tool for helping to find medically actionable results, and Berg et al.25 explored this topic in a 2011 commentary paper. Due to prohibitive costs of running GWAS for large populations, asymptomatic individuals who have family members who have developed a disease should be targeted. This achieves two goals: it helps to screen individuals of interest and builds sample cases of potential disease-causing variants. The screening for public health purposes also allows for a modification of protocol that is not practical with personalised cancer therapy. Any statistically driven test must balance sensitivity and specificity to avoid false-positives. For individual cancer treatment, approaches must have a high sensitivity; however, when performing these tests for public benefit, the scan can maximise specificity and minimise false-positive results, all at the expense of sensitivity. This will generate large numbers of variants, but these should all be approached with caution as the results have an increased likelihood of being a false-positive. This establishes a clearer picture of the breadth of possible variants for the disease.
The challenge then arises as to whether these increased findings should be acted upon, keeping in mind that many variants may be false-positives. To solve this, Berg et al.25 proposed removing incidental findings from these screenings. Three primary bins based upon the clinical implications of the finding would be established: Bin 1 would contain variants with known clinical use, like BRCA1/2 mutations, where there is a clinically actionable option available; Bin 2 would contain variants where there is clinical validity, but no strong clinically actionable options. Examples here would be prion diseases, Huntington’s disease, amyotrophic lateral sclerosis, and APOE gene polymorphisms. Bin 2 would also be subdivided into three subcategories of low, medium, and high risk for incidental information based on the patient’s preference. This allows people to opt-in for receiving fatal, degenerative disease results like amyotrophic lateral sclerosis or Huntington’s disease. Bin 3 is designed as a ‘catch all’ for findings with unknown clinical implications. However, it should be kept in mind that this is a proposed method for using GWAS as a public health tool and is not in actual practice. By being at the opposite end of the translational medicine curve, public health takes the longest to integrate the innovation developed by bioinformatics, so the fact that GWAS adoption is still in the discussion phase is not surprising.
DISCUSSION
The omics influence on clinical practice is at a point of transition and the surveillance sequencing work is an example of when it has been easily integrated into public health and clinical practice. There are also scenarios where efforts are just beginning, as explored with the cancer studies that use GWAS. Therefore, is it prudent to start performing genomic sequencing for individuals to identify potential health hazards? Doing so would allow identification of genetic variants that increase a patient’s chances of developing common diseases or cancers. Interventions could begin earlier, helping reduce risk through behaviour modification (healthier diet or increased exercise) or leading to placement of patients on drug therapies. On the surface, it appears that this additional layer of information would have similar healthcare cost savings as the practice of preventative medicine. If it is possible to prevent or delay the onset of serious disease, major medical expenditures can be avoided. GWAS and WES are some of the most effective tools we have that allow implementation of this, but there are downsides to consider, prompting the question: is it prudent to deploy these strategies at a population level?
There are four major criteria that should be considered before deploying a genetic screening programme to identify disease risk for a population. The first consideration should be: is the information captured from the genetic screening better at predicting the disease than traditional phenotypic methods? The second criterion is that a cost-effective intervention needs to be currently available for the disease with an increased risk profile. Benefits are drastically reduced if there are little-to-no options available to the patient. Also, does simply being aware of the risk automatically justify the cost of the screening test? Cost is a central point for the third criterion, namely, are these genetic screening methods more cost-effective than other population-level interventions? Deploying strategies like adding ‘sin taxes’ to fizzy drinks and cigarettes or creating national advertising campaigns may be more cost-effective than screening tests. The final consideration is: does the knowledge of genetic risk enhance behaviour change? If an individual is aware of an increased risk of diabetes, will they start exercising more and improve their diet? As it stands now, there is a lack of screening tests that can meet all four of these criteria.26
GWAS have helped advance our understanding of the genome in many ways, but as far as identifying variants with increase odds ratios for predicting disease, results have not been encouraging. Most odds ratios for disease uncovered through GWAS have been in the range of 1.1–1.6.25,27,28 It can be difficult to find novel loci with GWAS, as major variants in the genome that have significant odds ratios have often already been found through other methods, as seen in some studies on BRCA mutations.29,30 GWAS have been effective at identifying minor influences, but less successful in finding the major drivers for disease. When contrasting GWAS results and the traditional medical practice of focussing on family history and environmental factors, results are a marginal improvement at best. Looking at genetic variants for Type 2 diabetes mellitus was only a slight improvement in predictive power over other variables like age, BMI, and sex.31 In other studies, genetic variant tests proved less predictive for coronary heart disease than traditional predictive factors of age, blood pressure, cholesterol, triglycerides, cigarette use, and diabetes.32 Therefore, the first major criterion listed for consideration to deploy widescale genome screening has not been cleared yet.
The second criterion is more of a case-by-case scenario, but it follows previously established ethical standards for conducting the screening test to begin with.33 Take for example prostate-specific antigen screening. Identifying the presence of prostate-specific antigen indicates an odds ratio of 0.52–0.67 for developing prostate cancer;34 however, it would involve screening 1,500 males to prevent a single death, and would result in 80 out of those 1,500 having to undergo unnecessary major surgery.35
How well does knowledge of genetic predisposition to disease drive behaviour change? The resulting behaviour changes seen so far have been small and lack staying power.36 When informed of increased genetic risk for lung cancer, cigarette smokers did reduce the number of cigarettes smoked per day, but this effect did not last >6 months.37 There is also a concern about ensuring clear communication about genetic risks. Miscommunicating genetic risks can have a deleterious effect on an individual’s belief that they have the capacity to change behaviours.38 The effectiveness of helping people quit smoking by informing them of genetic risks has a very long way to go to match the success of other behavioural modification techniques. It has been documented that these population-based strategies operate at more efficient margins than high-risk strategies,39 as taken by predictive genomic medicine. The high-risk strategy approach is seeking out those who are at greatest risk of developing a disease for treatment; these individuals are found towards the end of a normal distribution curve, so focussing on the higher numbers closer to the top of the curve can have a larger impact overall.
From a population standpoint, involving omics-derived data may not be the best use of our resources at present. Hall et al.26 also make the astute point that with the lack of major cost-effectiveness of population genomic screening, the message may be subverted by industry. This has occurred in the past when the tobacco industry tried to promote genetic causes of disease to exonerate smoking as a cause;40 however, the same barriers are not present when viewed at the individual level. The Mount Sinai study19 demonstrated that the burden of cost-effectiveness is reduced when working with patients rather than populations. This is not to suggest that these interventions are cheap, as they remain very expensive, and the cost is prohibitive for the vast majority of people; continued innovation from bioinformatics and industry will help to reduce the cost over time. Improved understanding and filtering of results from GWAS, or a future iteration of the technology, may help to reach the point where population genomic screening will be achievable, but it still eludes our grasp for now. The intersection of omics and precision medicine in the future will rely on several components, including advanced bioinformatics, the merger of multi-omics technologies, big health data, and deep machine learning to extract novel insights.
CONCLUSION
Public health surveillance work spotlights the potential of omics-driven innovations. However, with other areas like cancer, it is either just being developed or the ethics and methods are still being debated. While omics-based methodologies have not yet been implemented into widespread practice, that time is quickly approaching. A constant theme with genomics has been learning about increasing levels of complexity. Before the completion of the Human Genome Project, non-coding sections of DNA were thought to simply be ‘junk DNA’, implying these sections were not important or worthy of investigation. However, now the name has become a misnomer due to the discovery of features like transcription factors among these sections. Such is the nature of genomics, and, in turn, precision medicine; for every discovery that helps to simplify our understanding, there will be many more that add layers of complexity. The process of precision medicine has been akin to solving one Gordian knot sealing a box, only to find another potentially more complex one inside. These omics-derived tools, in concert with others, can help researchers and clinicians tease apart the next layer in that knot, by which progress is made. It is this progress that may, one day, deliver on the promise of precision medicine.