Venous thromboembolism (VTE) risk assessment is a major challenge in cancer outpatients treated with chemotherapy, as its development may result in increased morbidity and treatment delays, with detrimental effects on the overall outcome and quality of life.1 Although appropriate thromboprophylaxis could substantially improve patient care, it still remains sub-optimal and rather empiric, as highlighted at the European Society for Medical Oncology (ESMO) 2017 congress by Scotté et al.,2 who stressed that the uncertainty regarding whether to use thromboprophylaxis reflects the absence of clear guidance.
Indeed, current guidelines do not recommend routine prophylaxis for VTE primary prevention in ambulatory cancer patients receiving chemotherapy, especially those under adjuvant and/or hormone treatment.3,4 Nonetheless, as stated in the 2017 updated ESMO Clinical Practice Guidelines on supportive care, thromboprophylaxis ‘may be considered in high-risk patients receiving palliative chemotherapy for locally advanced or metastatic disease.’5 This statement emphasises how selecting patients for prophylactic anticoagulation is perceived as a growing necessity in cancer management, fostering the demand for risk assessment models (RAM).
Presently, pre-chemotherapy assessment of individual VTE risk is based on the Khorana Score (KS),6 the sole validated RAM for cancer outpatients. However, even though KS is a user-friendly predictor based on routinely available variables, it strongly depends on tumour type and does not consider certain cancer types associated with VTE (e.g. brain tumours) or treatment-related factors. Therefore, its major limitation is that >50% of patients fall into an unclear group.7,8 Accordingly, expanded RAM, including anti-cancer drugs or biomarkers, have been proposed, but a recent comparative analysis objected to the use of any of the available models.9
These considerations were the subject of discussion within the ESMO 2017 congress, where we presented a novel approach to developing VTE classifiers and a web service supporting the oncologists in risk assessment. The rationale was based on our recent demonstration that a machine learning approach based on multiple kernel learning (MKL), combining support vector machine algorithms and random optimisation, is capable of exploiting significant patterns in routinely collected demographic, clinical, and biochemical data.10,11 This results in a substantial advantage over traditional RAM (e.g. the KS, as reported in Figure 1) which are based on the arbitrary assignment of a score according to association analyses.
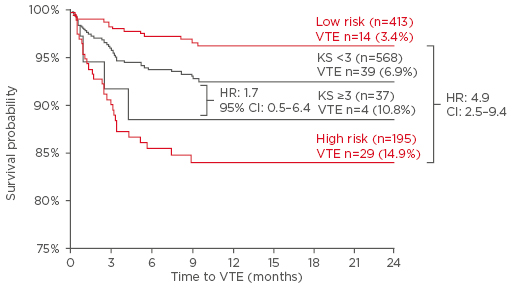
Figure 1: Kaplan-Meier curves of venous thromboembolism-free survival of chemotherapy-treated ambulatory cancer patients.
Comparison between patients stratified into low or high risk using the Khorana Score (black lines) or the VTE RISK score (red lines).
CI: confidence interval; HR: hazard ratio; KS: Khorana Score; VTE: venous thromboembolism.
The VTE RISK Web Predictor is a web service with a graphical interface of risk model, based on a set of two MKL predictors (Figure 2). This service has two main components: a web interface (client) and a decision server. The web interface allows users to insert values for VTE risk evaluation and displays a prevision message. The interface is designed to help oncologists input data into the system. Hence, the client web form provides automatic suggestions and lists of options for the fields, and calculates values by using others provided by users. At the request of the user, the servlet contacts the decision server (i.e. the reasoning component that implements the kernel function), which computes the risk and returns estimates in the client form, even in the event of missing data, because the MKL allows some empty values in input form. This is represented by a binary value (0/1) achieved by both predictors according to a voting on the positive class.
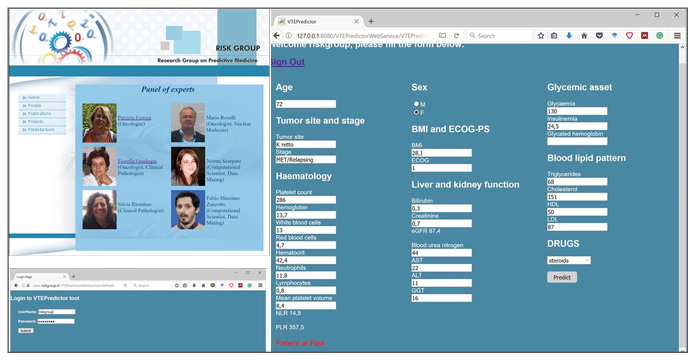
Figure 2: RISK Web Predictor.
Client form response12 (www.riskgroup.it).
In the context of developing predictive models, the use of MKL does not only provide an advantage over traditional models but, most importantly, retains several advantages in a perspective of precision medicine, with possible recalculation of updated data over time. The web interface will be publicly available shortly. We welcome the opportunity to invite interested researchers in its clinical application.