INTRODUCTION
Radiomics has been proven effective for the characterisation of primary prostate cancer (PCa).1,2 However, the limited interpretability of the proposed models represents one of the major limitations in this field.3,4 This study investigated 68Ga-prostate-specific membrane antigen (PSMA) PET radiomics for the prediction of post-surgical International Society of Urological Pathology (ISUP) grade in patients with primary PCa, ensuring model interpretability.
MATERIALS AND METHODS
Forty-seven patients with PCa were examined with 68Ga-PSMA PET at the authors’ institution. Those patients were enrolled in this study prior to radical prostatectomy. Images were acquired using either PET/MRI or PET/CT. ISUP grade was available at both biopsy and radical prostatectomy for all patients. A radiologist manually segmented the whole prostate on PET images using the co-registered CT or MRI for anatomical localisation on 3D Slicer software (Brigham and Women’s Hospital, Boston, Massachusetts, USA).5 The whole prostate was used as volume of interest (VOI) to avoid the limitations of radiomics for small volumes.6 VOIs were normalised, resampled, and discretised. A total of 103 image biomarker standardisation initiative-compliant, radiomic features (RF) were extracted using PyRadiomics (Python Software Foundation, Beaverton, Oregon, USA).7 RFs were harmonised with the ComBat method8 to control for the scanner effect, and selected using the minimum redundancy maximum relevance algorithm. Combinations of the four most relevant RFs were used to train 12 radiomics machine learning models for the prediction of post-surgical ISUP ≥4 versus ISUP <4 that were validated by five-fold repeated stratified cross-validation. To ensure that results were not driven by spurious associations, two ad hoc control models were generated. The first one had SUVmax and VOI volume as input (radiomics baseline), while the other was made by setting to zero all voxel values prior features extraction (PET zeros). Balanced accuracy, sensitivity, specificity, and positive and negative predictive values were collected. The performance of the best developed model was compared with that of ISUP grade biopsy.
RESULTS
ISUP grade at biopsy was upgraded in 9 out of 47 patients after prostatectomy, resulting in a balanced accuracy of 85.9%; sensitivity of 71.9%; specificity of 100.0%; positive predicted value of 100.0%; and negative predictive value of 62.5%. The best performing radiomic model yielded a balanced accuracy of 87.6%; sensitivity of 88.6%; specificity of 86.7%; positive predicted value of 94.0%; and negative predicted value of 82.5%. All radiomic models trained with at least two RFs (grey level size zone matrix; zone entropy and shape; least axis length) outperformed the control models. Conversely, no significant differences were found for radiomic models trained with two or more RFs (Mann–Whitney U test; p>0.05). See Table 1 for a detailed report of all the generated models’ performance.
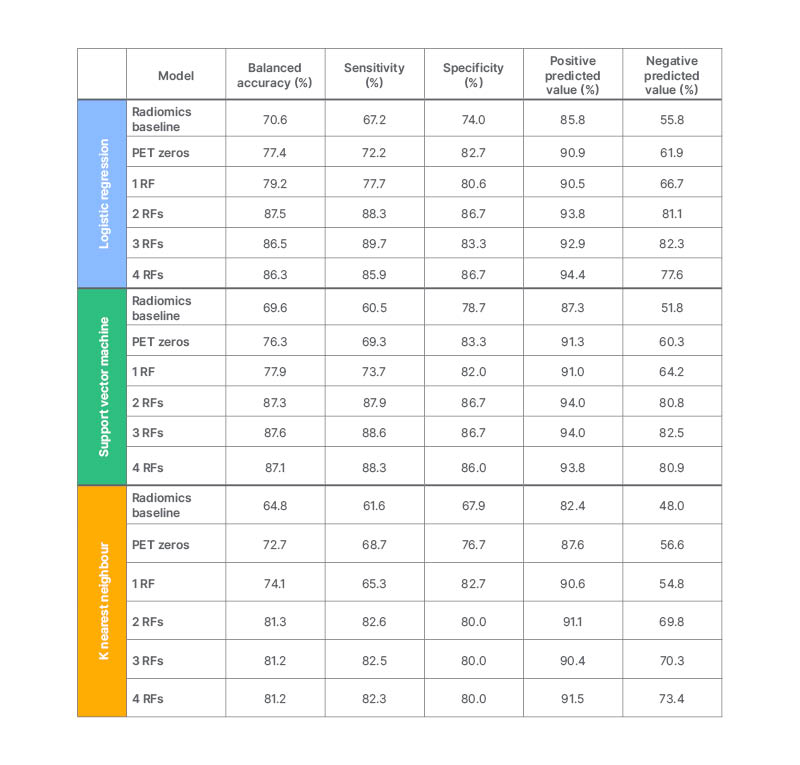
Table 1: Performance of the generated models.
RF: radiomic features.
CONCLUSION
These findings support the role of 68Ga-PSMA PET radiomics for the accurate and non-invasive prediction of post-surgical ISUP grade. Future multicentre studies will be needed to establish with certainty the accuracy and reproducibility of the radiomic signature proposed here.