Abstract
A high-throughput artificial intelligence-powered image-based phenotyping platform, iBiopsy® (Median Technologies, Valbonne, France), which aims to improve precision medicine, is discussed in the presented review. The article introduces novel concepts, including high-throughput, fully automated imaging biomarker extraction; unsupervised predictive learning; large-scale content- based image-based similarity search; the use of large-scale clinical data registries; and cloud-based big data analytics to the problems of disease subtyping and treatment planning. Unlike electronic health record-based approaches, which lack the detailed radiological, pathological, genomic, and molecular data necessary for accurate prediction, iBiopsy generates unique signatures as fingerprints of disease and tumour subtypes from target images. These signatures are then merged with any additional omics data and matched against a large-scale reference registry of deeply phenotyped patients. Initial applications targeted include hepatocellular carcinoma and other chronic liver diseases, such as nonalcoholic steatohepatitis. This new disruptive technology is expected to lead to the identification of appropriate therapies targeting specific molecular pathways involved in the detected phenotypes to bring personalised treatment to patients, taking into account individual biological variability, which is the principal aim of precision medicine.
INTRODUCTION
Over 52% of health system executives expect artificial intelligence (AI) tools for medical imaging to have a significant effect on their organisations in the next 5 years, according to a November 2018 report from the Center for Connected Medicine.1 At this time, nowhere in medicine has the impact of AI been more disruptive than in medical imaging. Many medical image equipment manufacturers are already incorporating various AI tools in their product offerings. Early computer-aided diagnostic applications have been used to assist radiologists in image interpretation and the detection of abnormalities in CT and MRI scans since the 1980s, but the use of these techniques were limited by high rates of false positives.2 In recent years, powerful AI algorithms using supervised learning based on neural networks, have vastly improved the accuracy of computer-aided detection. These new algorithms require vast amounts of manually annotated images for training. To date, early efforts are limited and have been able to assemble fully curated registries of a few thousand images. The small scale of these registries has limited the predictive power of the AI tools for precision medicine.
The next frontier in AI is unsupervised learning, more akin to actual human learning, whereby the system learns hidden patterns in the data by itself without explicit prior labelling. Such unsupervised approaches will be critical to the widespread adoption of AI for precision medicine.3
iBiopsy® (Median Technologies, Valbonne, France) is a novel, image-based, high-throughput phenotyping platform, currently under validation, which combines unsupervised, or predictive, learning for the automated detection and characterisation of phenotypic abnormalities extracted from medical images with real-time similarity search across large libraries of previously classified disease signatures. This new disruptive technology is expected to lead to the identification of appropriate therapies targeting the specific molecular pathways involved in the detected phenotypes to bring personalised treatment to patients, considering their individual biological variability, the principal aim of precision medicine.
THE CHALLENGE OF PRECISION MEDICINE
The Precision Medicine Paradigm
The Precision Medicine Initiative launched by USA President Barack Obama in January 2015 signalled a paradigm shift in healthcare, with an evolution away from the ‘one-size-fits-all’ approach to disease prevention and treatment strategies designed to meet the needs of the average person, towards a personalised treatment approach based on individual biology. Dr Francis Collins, who led the Human Genome Project, defines precision medicine as involving prevention and treatment strategies that take individual variability into account; in this sense, precision medicine has been vastly improved by the development of large-scale biologic databases, powerful methods for characterising patients, and computational tools for analysing large sets of data.4
Precision medicine relies on the availability of useful biomarkers, defined as ‘a characteristic that is objectively measured and evaluated as an indicator of normal biological processes, pathogenic processes, or pharmacological responses to a therapeutic intervention.’5
There is a need to identify which patients are likely to derive the most benefit from targeted therapies. This may include merging information from imaging, pathology, blood biomarkers, clinical data, genomic, and proteomic data into a final treatment decision.
Limitations of Electronic Health Record Systems for Precision Medicine
To meet the goals of precision medicine, large collections of detailed patient data registries (CDR) will be necessary to assess which patients may respond to which treatment and to predict outcome. To assemble such real-world evidence in advance, directly from providers, a very popular approach has been to mine data already collected from various electronic health records (EHR).6 The problem with EHR approaches is the reliability, quality, and validity of the data collected.7 The main problem is, as stated by Prof John Quackenbush, Dana Farber Cancer Institute, Harvard T.H. Chan School of Public Health, Harvard University, Boston, Massachusetts, USA: “EHR are not designed for treating patients, not designed for biomedical research, they’re designed for getting paid.”
In addition to quality and reliability issues, the current IT infrastructure is inadequate to store, process, and analyse the mass of omics data, such as genetic, pathology, and radiology data, produced by modern hospitals today, which are critical to the implementation of precision medicine approaches. The conclusion of a recent industry report by KLAS, an independent research group, was that precision medicine is too complex for EHR.8
The Promise of Medical Imaging for Precision Medicine
Medical images possess valuable diagnostic and prognostic information that can be mined through advanced image processing and data analytics methods. Computer-aided detection tools using imaging biomarkers were initially introduced in the 1980s9 to assist in the detection of abnormalities in medical images. While often quite sensitive, such methods lacked specificity and often required substantial manual preprocessing by skilled technicians.
The latest progress in computational power has given rise to a recently developed field of research called ‘radiomics’. Radiomics, which was first formally introduced in 2012,10 employs state-of-the-art machine learning techniques to extract and study large quantities of imaging data, such as features from radiological images; these data are used to decode underlying genetic and molecular traits for decision support. In radiomics, medical images that hold information on tumour pathophysiology are transformed into mineable high-dimensional data. This information can be harnessed through quantitative image analyses and leveraged via clinical-decision support systems. West et al.11 recently showed that the radiomics analysis on CT images of treatment-naïve hepatocellular carcinoma can reliably identify the expression of specific genes that confer chemoresistance to doxorubicin.11 This study may help tailor future treatment paradigms via the ability to categorise hepatocellular carcinoma tumours on the genetic level and identify tumours that may not have a favourable response to doxorubicin-based therapies.11 Additionally, a recent study showed that radiomics signatures can also define the level of lymphocyte infiltration of a tumour and provide a predictive score for the efficacy of immunotherapy in the patient.12
Radiomics and the Curse of Dimensionality
Like any high-throughput data-mining field, radiomics is subject to the ‘curse of dimensionality’,13 which was coined by mathematician Richard Bellman in 1961.14 The curse of dimensionality refers to various phenomena that arise when analysing and organising data in high-dimensional spaces (often with hundreds or thousands of dimensions) that do not occur in low-dimensional settings. The common theme of these problems is that when the dimensionality increases, the volume of the space increases so fast that the available data become sparse. This sparsity is problematic for any method that requires statistical significance.13 To obtain a statistically sound and reliable result, the amount of data needed to support the result often grows exponentially with the dimensionality (also known as the Hughes Phenomenon).15 At the same time, the quality of a machine learning model is closely tied to the complexity of the model or the number of dimensions. As the number of dimensions increases, the performance first increases, then rapidly decreases as new features are added. The lack of scalability of radiomics models has resulted in the inability to generalise results from many of the early studies to large populations. The curse of dimensionality is a major problem for all omic data. The nature of microarray data and its acquisition means that it is subject to the curse of dimensionality, the situation where there are vastly more measurable features (e.g., genes) than there are samples. Reductionist analysis of high-dimensional microarray data can lead to feature selection approaches that are liable to extreme type I error (false positives) and will not identify enough features to provide for individual differences within the patient cohort.16 Current radiomics studies suffer from the effects of similar reductionist approaches by seeking to select a few representative features from a large set of initial features. Unfortunately, the rapid growth in popularity of this immature scientific discipline has resulted in many early publications that miss key information or use underpowered patient datasets, without production of generalisable results.17
The predictive potential for systems biology, especially when applied to medicine, is the ability to capture not just the similarities between individuals in a cohort but the differences between them, enabling individualisation of patient treatments. It is widely predicted that novel deep learning methodologies derived from the latest developments in AI will help bring precision medicine into clinical practice.18
A fundamental problem in current translational radiomics research is the traditional paradigm of sifting through large numbers of features to identify a select group of markers to use prospectively for the diagnosis or prediction of therapies or outcome. If the potential of state-of-the-art imaging technologies is to be fully exploited for translational research and the individualisation of cancer therapies in particular, this paradigm needs to fundamentally change. Rather than striving for simplification, the goal of radiomic analyses should be to develop analytic approaches that use multidimensional datasets and embrace the complexity of genomic data for personalised medicine.
THE ARTIFICIAL INTELLIGENCE REVOLUTION
AI is basically machine perception or the power to interpret sensory data. Humans have the innate ability to recognise certain patterns, for example, when humans see two pictures of two different but similar-looking individuals, they can recognise that they are mother and daughter. Also, humans can hear two voices and recognise that the people talking have the same accent. This is a much more complex problem for a computer, but it is made possible through supervised learning computer algorithms, in which the algorithm is trained by being given thousands of examples of what you would like it to recognise. This technology is useful in voice and facial recognition software and many other applications, including recognition of features, such as tumours, in medical images. With the use of deep learning, subtle changes can be detected with much greater accuracy as compared to human analysis and the new algorithms will greatly enhance our ability to accurately detect and interpret imaging biomarkers.
Deep learning is a subset of machine learning. Its foundation was laid by Geoffrey Hinton and Yan LeCun,19 one of Hinton’s PhD students in the mid-1980s, although the mathematical principles reach as far back as the 1960s. The full potential of those methods available could only be realised following the exponential increase in computational power and the introduction of powerful and inexpensive graphics processing units (GPU).
AI is rapidly transforming the field of medical imaging and leading organisations are incorporating machine learning with the objective of improving physician decision- making and supporting the aim of individualised diagnosis and treatment of precision medicine.20 Deep learning has become extremely popular again in medical imaging since 2013, when new models based around convolutional neural networks substantially outperformed earlier systems for tasks, such as tumour classification and detection.20 Derived from the field of computer vision, convolutional neural networks are ideally suited for the large-scale analysis of medical images.
The First Generation: Supervised Machine Learning
The first major advances in the integration of AI in medical imaging involved supervised deep learning systems. The deep learning systems selected a set of features based on training with prior labelled datasets. The performance of such systems for basic classification tasks, such as segmentation with so-called deep neural networks (DNN), often matched or even exceeded that of trained radiologists.20
Supervised machine learning can be used effectively for anatomic structure segmentation.21 A deep learning model has also been used to train an algorithm to label individual voxels (pixels in three-dimensional space with added depth) within a liver CT scan, based on whether they are normal tissue or lesion. The accuracy of segmentation was reported at 94%, with each volume taking 100 seconds to process.22 While the accuracy of supervised learning models may be impressive, classification performance is closely tied to the combined dimensional complexity of the model and the large labelled dataset required for effective training. In most real-life situations, such large labelled datasets are generally unavailable. The total number of datasets may be limited, and the prior labelling may be very labour intensive or simply not known.
Second Generation: Unsupervised Machine Learning
“The next AI revolution will not be supervised,” argued the current Director of AI Research at Facebook, New York City, New York, USA, Dr Yan LeCun, at a recent AI Seminar.23
While fully unsupervised machine learning algorithms have been used in a variety of fields, the application of this technology to medical image analysis has been limited to fairly simple applications, such as image segmentation.24 Most unsupervised methods use algorithms that are entirely different from their supervised counterparts. Most of them fall in the category of clustering methods, the most common being the k-means clustering. Clustering methods are even older than deep learning methods, with their origin dating back to the 1960s with the publication of the monograph ‘Principles of Numerical Taxonomy’ by Sokal and Sneath in 1963.25 Just like DNN, clustering methods have become increasingly popular since the advent of fast processors to handle the complex computational requirements. Recently, a variety of new clustering methods have been introduced, one the most popular being the density peaks clustering method,26 introduced in 2014; this method is widely used for the clustering of gene expression microarray data.27
Another type of unsupervised machine learning network is referred as a restricted Boltzmann machine (RBM). The RBM unsupervised feature learning model has been applied to a number of tasks, including automatic detection of microcalcifications in digitised mammograms. It was used to automatically learn the specific features which distinguish microcalcifications from normal tissue, as well as their morphological variations. Within the RBM, low level image structures that are specific features of microcalcifications are automatically captured without any appropriate feature selection based on expert knowledge or time-consuming hand-tuning, which was required for previous methods.28
Transfer Learning
Training a DNN from scratch is difficult because it requires a large amount of labelled training data and a great deal of expertise to ensure proper convergence. A promising alternative is to fine-tune a DNN that has been pretrained using, for instance, a large set of labelled data from another field of medical imaging.29 However, the substantial differences between medical images from different modalities may advise against such knowledge transfer; knowledge transfer from a certain type of CT image, such as chest CT to abdominal CT, is more likely to succeed in the retraining of a DNN.30 However, this does not address the fact that large numbers of labelled images of any kind are hard to find in medical imaging. One of the largest collections available for research is the Lung Image Database Consortium image collection (LIDC-IDRI), which consists of diagnostic and lung cancer screening thoracic CT scans with annotated lesions. Seven academic centres and eight medical imaging companies collaborated to create this dataset, which contains 1,018 cases. The Radiological Society of North America (RSNA) has started to recruit volunteer radiologists at its main annual meeting to address the problem by manually labelling some random images for future research use. A main challenge with weakly supervised learning in medical imaging is that most works are proofs of concept on a single application, such as segmentation. This makes it difficult to generalise the results and gain insight into whether the method would work on a different problem. A review of >140 papers on transfer learning and weakly supervised learning concluded that, while the usefulness of the approach was shown, there was little evidence of successful results at this time.31
Generative Deep Learning Models
Generative DNN, more specifically Generative Adversarial Networks (GAN), can produce synthetic models of specific phenotypes against which target phenotypes can be compared. Such models have been applied to low-dose CT denoising, medical image synthesis, image segmentation, detection, reconstruction, and classification.32 Relevant anomalies can also be identified by unsupervised learning on large-scale imaging data.33 GAN can be used to increase the size of training sample sets by generating synthetic images of lesions.34 Interestingly, such GAN generally outperform state-of-the-art discriminative deep learning networks for small to medium-size datasets, the typical situation in medical imaging.35
THE NEXT GENERATION: IMAGING PHENOMICS
Managing Complexity with Phenomics
The complexity of diseases such as cancer necessitates a paradigm shift in our approach to precision medicine using high-dimensional data derived from high-throughput next-generation technologies. Such a paradigm shift will impact researchers, clinicians, trialists, and pathologists alike. A deep phenotype-driven approach, building models of integrated genetic, genomic, epigenetic, and clinical datasets, which represent individualised disease phenotypes, will allow physicians to predict a disease’s clinical course.
In biology, the most widely accepted definition of the word phenotype is ‘the observable traits of an organism.’ The study of phenotype in medicine comprises the complete and detailed understanding of the spectrum of phenotypic abnormalities associated with each disease entity.36 With this knowledge in hand, physicians can decide whether any given sign or symptom is related to an underlying disease or is an isolated feature, a decision that may help to stratify treatments and make the correct prognosis.
The phenome is the manifestation of all possible interacting influences, it contains information about all epigenetic and post-translational modifications above and beyond genes as well as environmental influences.37 Phenotypes are often highly heterogeneous, with different subgroups having potentially very different causes; many are still based on diagnostic criteria defined a century or more ago, which potentially encompass similar phenotypes but have no similarity in molecular origin. This heterogeneity is one often-cited reason why molecular precision medicine approaches usually disappoint when applied to such diseases: if the phenotype used to select the subject includes multiple different diseases with different pathogenic mechanisms, the statistical power of the study disappears. Phenomics, through the analysis of detailed phenotypic data, provide an opportunity to elucidate the causal routes at play for specific genetic variants on a phenotype and may ultimately lead to better targets for the prevention of multiple conditions and complications.
In a phenomics approach, the data are collected in a ‘hypothesis-free’ mode; the only major consideration is having enough representatives of rarer phenotypes within a broadly unselected population. Accessing phenotypes with a population frequency >1% is a relatively easy reachable goal with a few thousand subjects enrolled.
In a phenomics-driven model, the depth of the phenotype can be extended to include any number of variables, such as immune response markers and epigenetic markers, such as methylation, immunochemistry markers, and molecular pathology to identify drugable molecular pathways. Already >80% of new drug candidates are identified through phenome- wide association studies (PheWAS) as opposed to genome-wide association studies (GWAS).
Molecular pathological epidemiology, which studies the effects of external and internal factors on the phenotypes of disease outcome, such as in cancer and non-neoplastic disorders, will also play a major role in precision medicine and phenomic approaches. With analyses of external and internal factors, bacteria, and immune cells in relation to the disease of interest, molecular pathological epidemiology analyses can provide new insights into environment-disease interactions.38
Imaging Phenomics
Imaging phenomics is the systematic, large scale extraction of imaging features for the characterisation and classification of tissue and disease phenotypes. Imaging phenotypes are highly effective tools for quantitatively assessing, classifying, and predicting disease processes and their severity. They can help define these precise subpopulations.
- Imaging phenomics may serve as a foundation for precision medicine surveillance of disease manifestation (occurrence, location, extent, and severity) and progression.
- Imaging phenomics can help identify molecular subtypes of tumours with specific outcomes. Tumour heterogeneity and other textural features extracted from medical images have been correlated with various tumour subtypes from histopathology and risk of recurrence.
- Imaging phenomics is a big data problem, requiring large parallel computing resources and advanced analytics. Imaging phenomics leverage the latest deep learning technologies that may be used to automatically identify which imaging features best predict a trait.
COMBINING IMAGING PHENOMICS AND ARTIFICIAL INTELLIGENCE: THE iBIOPSY PLATFORM
The iBiopsy platform is a tissue and disease high-throughput phenotyping platform specifically designed to acquire, index, and analyse thousands of individual image-based phenotypes to establish biological associations with high predictive accuracy. The key innovative features of the iBiopsy platform include an automated and fully unsupervised signature extraction and clustering engine and a real-time search and retrieval engine to match a target phenotype against a registry of reference phenotypes. When released in 2019, after receiving U.S. Food and Drug Administration (FDA) clearance, iBiopsy will deliver an easy-to-use solution, decoding image signatures from standard medical images, and is expected to revolutionise the way cancer and many other chronic diseases are diagnosed, treated, and monitored.
At its core, iBiopsy is designed to make holistic real-time interrogation of complex and voluminous data possible by using unsupervised predictive learning methodologies and big data analytic tools that process the data in high-dimensional space and capture all the biologic dimensions of a disease phenotype for an individual patient as a single fingerprint.
Content-Based Image Retrieval
Traditional medical image retrieval systems, such as picture archival systems, use structured data (metadata) or unstructured text annotations (physician reports) to retrieve the images. The content of the images cannot be completely described by words, and the understanding of images is different from person to person; therefore, a text-based image retrieval system cannot meet the requirements for the retrieval of massive images. In response to these limitations, content-based image retrieval (CBIR) systems using visual features extracted from the images in lieu of keywords have been developed and introduced in a number of fields, especially computer vision.39 CBIR systems, with the advantages of high retrieval speed, have been widely applied for medical teaching, computer-aided diagnostics, and medical information management. Yet another important and useful outcome of a CBIR is the possibility to bridge the semantic gap, allowing users to search an image repository for high-level image features. In radiology, CBIR systems are an ongoing field of research.40 Current CBIR systems perform global similarity searches (i.e., seeking to match an entire image to a set of reference images). In an image analysis environment, phenotypes may be understood as abnormal pathologies which are highly local in nature. From that perspective, an ideal CBIR performs in a manner analogous to a virus-checking software on a computer, detecting and indexing abnormal phenotypes.
Automated Image Processing
The CBIR system implemented by Median Technologies, uses patented algorithms and processes to decode the images by automatically extracting hundreds of imaging features as well as highly compact signatures from tens of thousands of three-dimensional image patches computed across the entire image. In addition to detailed phenotypic profiles, which can be correlated with histopathology, genomic, and plasmic profiles, the system generates a unique signature for each tile providing a fingerprint of the ‘image-based phenotype’ of the corresponding tissue (Figure 1). Using massively parallel computing methods, imaging biomarkers and phenotype signatures extracted from a target image are then organised into clusters of similar signatures and indexed for real-time search and retrieval into schema-less databases.
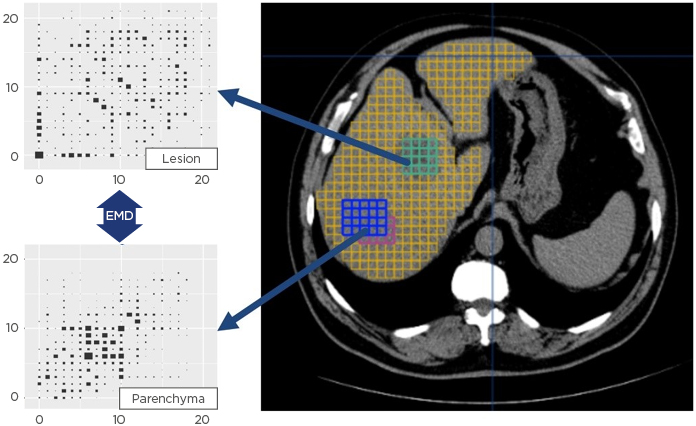
Figure 1: Unique image signature extraction for each tile provides a fingerprint of the matching tissue.
EMD: Earth mover’s distance.
iBiopsy Functionality
The iBiopsy phenotyping platform is based on three main engines: a signature extraction and indexing engine, a real-time search engine, and a predictive analytics engine that translates the results of the similarity search into a phenotype or outcome probability.
While at its core, iBiopsy has focussed on the complex issue of dealing with unstructured imaging data, which is a source of highly accurate and noninvasive quantitative biomarkers of disease processes, the platform is not limited to the handling and processing of medical images. iBiopsy is an ecosystem for big data analytics and can also accept pathology, molecular, genetic, and epigenetic data in the development of a comprehensive disease phenotype. iBiopsy is built on a highly scalable cloud-based framework capable of searching through petabytes of data in real time. It is designed to acquire, process, and cluster essentially any type of data, without the need to understand a priori the meaning of each data element.
Signature Extraction and Indexing
The image processing operations required for local content-based image feature extraction consist of two main tasks: firstly, tiling the images in a smaller volume-of-interest (VOI), typically a small cube, the size of which depends on the modality, the image resolution, and the purpose of the content-based query; secondly, performing feature extraction operations on the VOI.
As illustrated in Figure 2, the feature extraction engine performs totally unsupervised, automatic, and asynchronous extractions of features from the images, organises, and indexes them in a non-structured query language database based on a unique similarity metric.
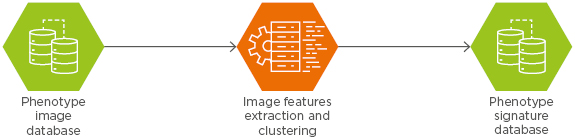
Figure 2: Feature extraction engine.
The result of the first phase is a series of clusters of phenotype signatures. Since the clusters are self-organising, their pathophysiological meaning is not readily apparent and requires further analysis. The characterisation of each cluster is typically performed by analysing representative samples and their respective correlate with histopathology. Eventually, after a series of iterations, the clusters are organised to correlate with distinct tissue subtypes identified by their signature similarity. The final number of clusters is not known a priori and depends on the heterogeneity of the underlying imaging phenotypes.
Real-Time Search Engine
The interactive search subsystem provides users with a query interface to the search engine where they can select or upload a query or example image tile and set metadata constraints for the search. The metadata may include the imaging modality (such as CT, MRI, or PET), voxel size, radiation dose in cases of CT imaging, or imaging protocol (e.g., T1 and T2) in cases of MRI. The presence of a contrast agent can also be included. A questionnaire may provide information related to patient data such as weight, size, prosthesis, previous local treatment, or prior disease conditions likely to affect biomarker evaluation. The search subsystem is then invoked to transform the user provided examples to a form suitable for query processing (i.e., performing any pre-processing and feature extraction operations on them) and then to determine the best matching signatures based on the content index. Finally, the interactive visualisation subsystem receives the identifiers of the best matching signatures and displays the corresponding VOI results. The analysis of the data is performed in real time with great flexibility using an optimised metric indexing image archiving system.
As illustrated in Figure 3, the Median Technologies, CBIR system performs real-time similarity searches of indexed phenotype signatures against one or more target patient signatures in the CDR. As a result of the search, a series of matching signatures are returned together with their associated metadata. In addition to returning actual images, the query returns a series of summary statistics, which may be used to predict diagnosis or prognosis based on CDR. The larger the CDR, the more accurate the prediction.
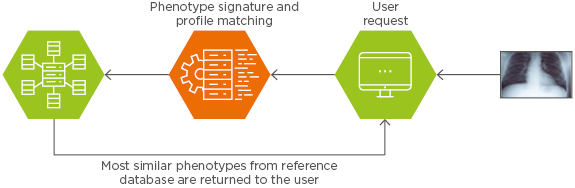
Figure 3: Real-time similarity search engine.
Predictive Analytics Engine
The third element of the iBiopsy platform is the predictive analytics or inferencing engine, which uses various AI algorithms to make a prediction to a clinical question asked by a user, such as identifying the specific tumour subtype, and make a prognosis about outcome based on patients in the CDR with similar subtypes.
A unique strength of the iBiopsy platform is the fact that is built entirely hypothesis-free as opposed to fit-for-purpose (i.e., it is not configured to answer a predefined set of questions, but rather it can answer any number of questions that may not have been considered at the time of design). In that sense, iBiopsy is very similar to an internet search engine, except that it operates both on textual metadata and on data automatically extracted and indexed from raw images. To make a final prediction, iBiopsy uses an ensembling strategy whereby independent algorithms reach a result and the final prediction is obtained by consensus between the methods for higher reliability.
Current Status and Future Challenges
The validation of the iBiopsy platform as a robust and reliable image-based high-throughput phenotyping platform for large-scale deployment in clinical research and routine practice is ongoing and following a multistage process.
The first stage involves the analytical validation of the platform for phenotype extraction and indexing. This mostly involves large scale tests of repeatability and reproducibility. Repeatability testing involves comparing results from image data generated within short intervals on the same patient in the same setting. Reproducibility testing involves testing the robustness of signatures under different acquisition conditions, with different equipment, technicians, and physicians. These tests have largely been completed and the results presented at major radiological and other medical conferences.41
The next phase involves qualifying the iBiopsy signatures against pathology and clinical outcome. For that purpose, large-scale data registries of images, pathology samples, genomic, and clinical data from patients with hepatocellular carcinoma are being assembled in the USA, Europe, and China with leading liver hospitals.
Initial results have also been presented on the challenging problem of noninvasively measuring the extent and severity of liver fibrosis in patients with nonalcoholic steatohepatitis (NASH).42 Such measures currently involve invasive and costly biopsies. In partnership with major pharmaceutical companies, the platform is currently being tested for the discovery of various novel biomarkers for the staging and prognosis of NASH.
FUTURE OPPORTUNITIES AND CHALLENGES
Imaging phenomics offers the opportunity to leverage advances in AI in the field of medical imaging for precision medicine by identifying patients with specific disease subtypes, which may be the focus of targeted therapies, and by predicting the outcome from these therapies. Unsupervised learning has the potential to fundamentally change the treatment paradigm for many cancers and chronic diseases: rather than base treatment on empirically derived guidelines, it is guided by big data analytics to predict treatment response, leveraging vast biobanks and clinical data registries. There have been great advances in machine learning approaches, which can build predictive models from very complex datasets incorporating imaging, genetics and genomics, and molecular data, as well as environmental and behavioural data. AI approaches can be used to explore and discover new predictive biomarkers from these datasets. This information can then be used throughout the clinical care path to improve diagnosis and treatment planning, as well as assess treatment response.
There are limitations with current AI solutions. A case in point is the recent collapse of MD Anderson Cancer Center’s ambitious venture to use the Watson cognitive computing system, developed by IBM, to expedite clinical decision-making around the globe and match patients to clinical trials. Launched in 2013, the project, while initially touted as revolutionising cancer care, never delivered actionable data to physicians and Watson was never used to treat patients at MD Anderson.43
To improve the predictive ability of AI-driven systems, models trained on vast and complex datasets are needed. AI can support the automated analysis of large and heterogeneous sources of data, increasing the diagnostic and prognostic value derived from image datasets alone. AI-based surveillance programmes can identify suspicious or positive cases for early radiologist review and extract information not discernible by visual inspection.
CONCLUSION
Several challenges still need to be addressed before fully unsupervised medical imaging can be taken into clinical practice, especially the constitution of large clinical data registries and the development of a new ecosystem for handling, storing, and analysing petabytes of omics data. While regulatory, legal, and ethical issues may constrain the scope of AI for precision medicine, imaging phenomics in particular is expected to play an increasingly important role in translational medicine for the development and validation of new therapies using phenome-wide approaches. The rapid adoption of AI-based approaches by the medical imaging community holds promise that imaging will continue its evolution, playing a pivotal role in enabling precision medicine to be widely realised in practice.